Business analytics involves leveraging data management, visualization, and predictive modeling to make informed decisions. I focus on data integration to guarantee quality and derive insights from various sources. Descriptive analytics examines historical data to monitor performance and identify trends, while predictive analytics uses statistical models to forecast future outcomes. Prescriptive analytics then suggests best actions based on past data. Effective business analytics incorporates techniques like regression analysis and machine learning. By understanding these components, we can optimize processes and make strategic decisions that enhance efficiency. Let's explore further how these elements work together to transform data into actionable insights.
Key Takeaways
- Business analytics involves analyzing historical data to identify trends and gain insights for informed decision-making.
- Predictive modeling uses historical data and statistical techniques to forecast future trends and behaviors.
- Data management ensures the quality and integrity of data used in analytical processes.
- Visualization transforms raw data into visual formats like charts and graphs for easier interpretation.
- Prescriptive analytics suggests optimal actions based on past performance data and advanced algorithms.
What Is Business Analytics?
Business analytics is the practice of using data analysis and statistical models to solve business problems and inform decision-making. As a Data Analyst, I focus on extracting valuable insights from data.
Using descriptive and predictive analytics, I uncover trends that guide strategic decisions. Predictive models, for instance, help anticipate future outcomes, while prescriptive analytics suggests actionable steps. These methods are indispensable across industries, optimizing operations and preparing for future challenges.
Descriptive analytics provides a historical view, enabling us to understand past performance. By leveraging statistical tools, I guarantee each decision is data-driven, enhancing business efficiency.
The global market for business analytics is booming, highlighting its critical role in modern business strategy.
Key Components of Business Analytics
Fundamentally, the key components of business analytics—data management, visualization, predictive modeling, data mining, and optimization—transform raw data into actionable insights. Data management guarantees data quality and accessibility, while visualization converts complex data into easily understandable reports. Predictive modeling and data mining uncover patterns and trends, aiding in decision-making. Optimization refines processes for efficiency.
Component | Function | Outcome |
---|---|---|
Data Management | Guarantees data quality | Reliable data for analysis |
Visualization | Converts data into visuals | Easily understandable reports |
Predictive Modeling | Identifies future trends | Proactive decision-making |
Data Mining | Extracts hidden patterns | Actionable insights |
Optimization | Refines processes | Enhanced operational efficiency |
Combining technical expertise and communication skills is essential to presenting findings effectively and driving innovation.
Descriptive Analytics Overview
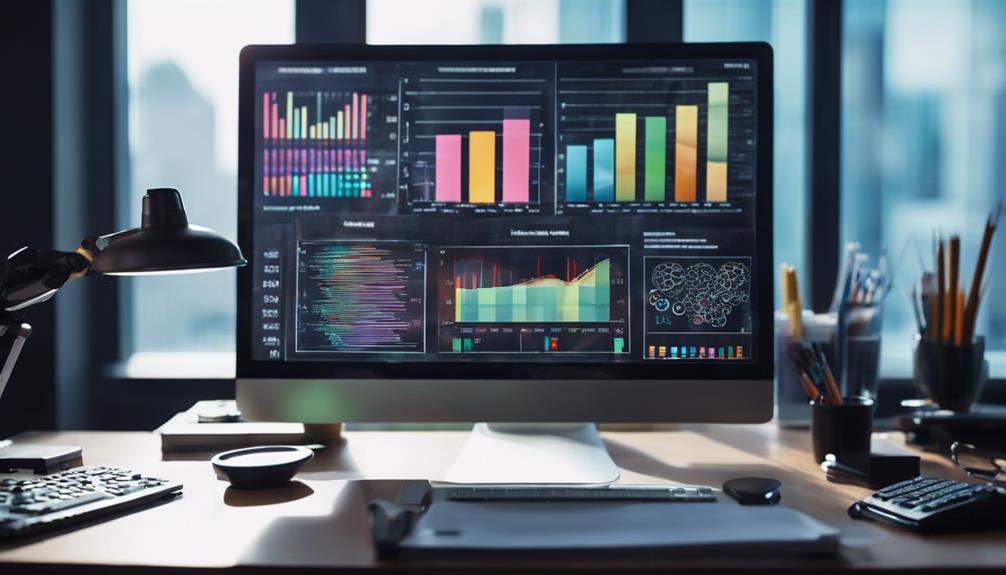
Descriptive analytics provides a foundation for understanding the historical performance of a business by meticulously analyzing past data. By examining historical data, I can identify past trends and patterns that are essential for tracking key performance indicators (KPIs).
This type of analytics is essential for reporting and dashboard creation, allowing for effective monitoring of business performance. Through descriptive analytics, I can interpret data to generate valuable insights, paving the way for informed decisions.
Predictive Analytics Explained
When I explore predictive analytics, I focus on how businesses use historical data and statistical modeling techniques to forecast future trends.
These methods enable data-driven decision making, allowing companies to anticipate customer needs and market changes effectively.
Forecasting Future Trends
Predictive analytics uses statistical algorithms to forecast future trends by analyzing historical data and identifying patterns. By leveraging this approach, I can anticipate customer behavior and market trends, making proactive decisions that enhance strategic planning.
This method allows businesses to generate data-driven predictions that are vital for operational efficiency. By understanding past data, I can identify correlations and patterns, which helps in reducing costs and maximizing profitability.
Predictive analytics also empowers me to foresee potential risks and opportunities, enabling a more calculated and informed approach to business strategy. Ultimately, this robust analytical tool is indispensable for any organization aiming to stay ahead in a competitive market through innovative and efficient practices.
Data-Driven Decision Making
Leveraging historical data and statistical algorithms, predictive analytics allows businesses to make data-driven decisions by forecasting future trends and identifying potential risks. By analyzing trends and patterns, businesses can make informed decisions that enhance strategic planning and operational efficiency.
Predictive analytics is pivotal for sales forecasting, helping to anticipate demand and optimize inventory. In customer behavior analysis, it predicts purchasing patterns, enabling tailored marketing strategies.
Risk assessment benefits from predictive models by identifying potential risks before they impact the business. The accuracy of these forecasts depends on the quality of the historical data and the robustness of the statistical algorithms used.
Continuous validation and refinement of the models guarantee they remain relevant and effective in a dynamic market environment.
Statistical Modeling Techniques
Building on the importance of data-driven decision making, I now explore the statistical modeling techniques that form the backbone of predictive analytics. These techniques involve regression analysis, time series analysis, machine learning algorithms, and data mining.
By examining patterns and trends in historical data, predictive analytics enables businesses to forecast future outcomes accurately. Whether it's demand forecasting, risk assessment, or customer segmentation, these methods provide actionable insights.
For instance, regression analysis helps in understanding relationships among variables, while time series analysis tracks data over intervals. Machine learning algorithms enhance prediction accuracy by learning from vast datasets.
Together, these statistical modeling techniques empower businesses to innovate and stay ahead in competitive markets through precise, data-driven strategies.
Prescriptive Analytics Insights
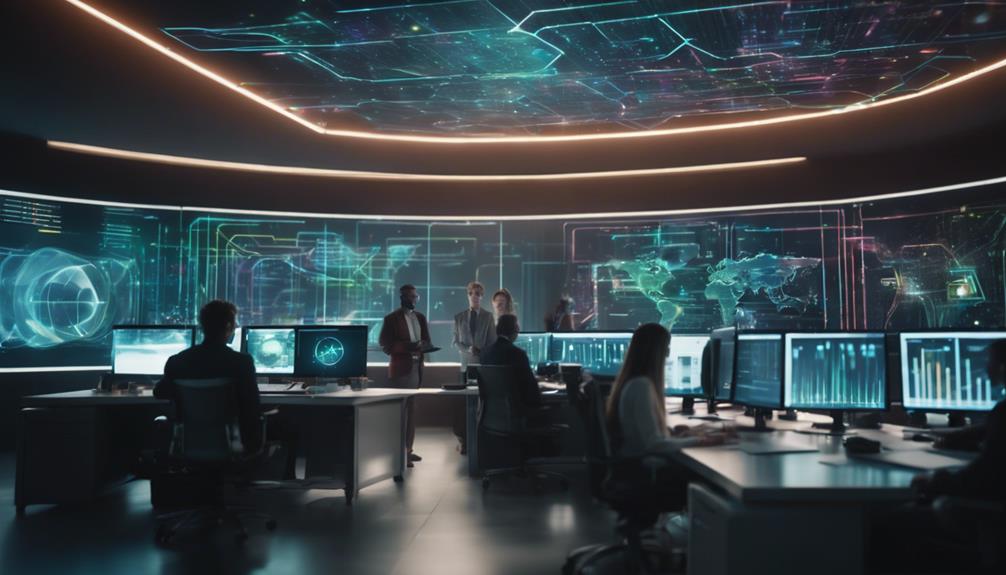
Prescriptive analytics delivers actionable recommendations that guide businesses towards best decisions by analyzing past performance data and leveraging advanced algorithms. It focuses on suggesting ideal actions to achieve desired outcomes. By using historical data, prescriptive analytics enhances decision-making and improves future results. Businesses can make informed decisions and boost operational efficiencies through these specific recommendations.
Here's a quick overview:
Aspect | Description | Benefit |
---|---|---|
Prescriptive Analytics | Uses past performance data | Provides ideal actions |
Decision-Making | Leverages advanced algorithms | Enhances informed decisions |
Recommendations | Suggests best courses of action | Improves future results |
Historical Data | Analyzes past trends | Guides decision-making |
Operational Efficiencies | Increases through actionable insights | Maximizes business outcomes |
Collecting and Integrating Data
When I collect data, I gather information from various sources like databases, spreadsheets, and online platforms.
Integrating these multiple sources involves merging, cleaning, and transforming raw data to create a unified dataset.
Using ETL processes, I can streamline data processing, ensuring accurate and reliable business analytics outcomes.
Data Collection Methods
Effective data collection methods in business analytics involve systematically gathering information from diverse sources like databases, surveys, social media, and IoT devices. I use these methods to guarantee a thorough dataset, essential for generating reliable insights.
The integration of data from disparate sources is streamlined through ETL (Extract, Transform, Load) processes, which convert varied data into a unified format. Data quality assurance is essential here, ensuring the accuracy, completeness, and consistency of the collected data.
Integrating Multiple Sources
Integrating multiple data sources is essential for consolidating diverse information into a unified repository, enabling thorough analysis and informed decision-making. By combining data from multiple systems, I can merge structured and unstructured data into a central database for a holistic view of business processes. Data integration tools clean, transform, and load data, ensuring its readiness for analysis and reporting. Effective data integration not only improves decision-making but also enhances insights and understanding of business operations.
Here's how different data sources contribute:
Data Source | Type | Purpose |
---|---|---|
CRM Systems | Structured | Customer relationship management |
Social Media | Unstructured | Sentiment analysis |
ERP Systems | Structured | Operational efficiency |
Web Analytics | Unstructured/Structured | User behavior tracking |
Statistical Models and Techniques
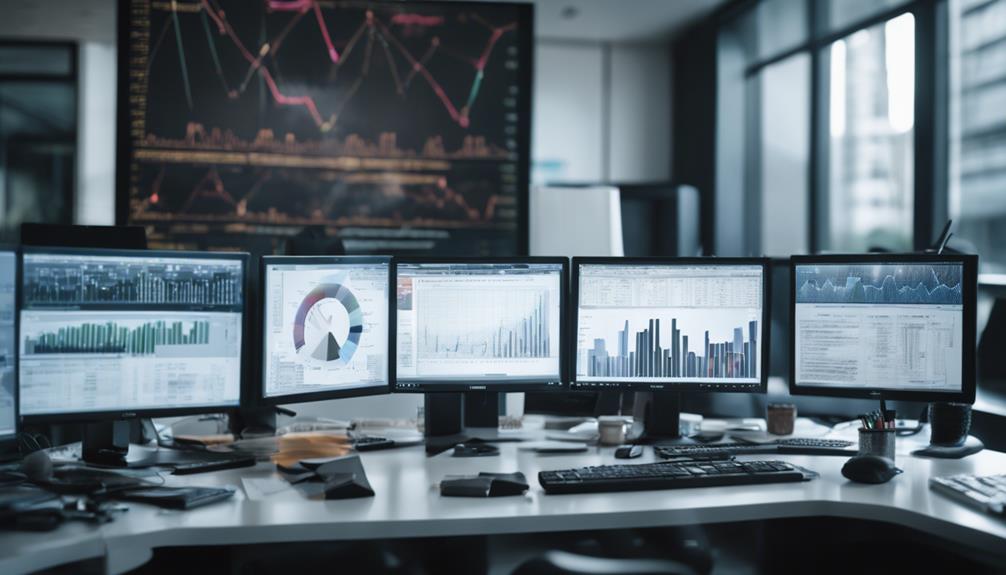
Statistical models like regression analysis, time series forecasting, and cluster analysis form the backbone of business analytics, enabling us to uncover hidden patterns and make data-driven decisions. These models analyze relationships between variables, allowing for accurate predictions and informed decision-making. Techniques such as correlation analysis, hypothesis testing, and ANOVA further refine our understanding by testing relationships and differences among variables.
Regression analysis helps identify trends, time series forecasting predicts future values based on historical data, and cluster analysis groups similar data points. By leveraging these models, businesses can pinpoint trends, detect outliers, and interpret data thoroughly, leading to actionable insights and innovative strategies. Embracing these techniques is essential for any forward-thinking organization aiming to thrive in today's data-driven world.
Frequently Asked Questions
What Is the Basic Business Analytics?
In basic business analytics, I focus on data collection, data visualization, and data integrity. Using analytical tools like Excel and Python, I perform descriptive statistics, predictive modeling, and statistical analysis, ensuring robust data governance and leveraging business intelligence from data warehousing.
What Are the Four 4 Types of Business Analytics?
Understanding the four types of business analytics is essential! Predictive modeling forecasts trends, Descriptive analytics explains past data, Diagnostic analysis identifies root causes, and Prescriptive methods recommend actions. Analytical tools, statistical techniques, and data visualization enhance business intelligence.
What Are the Basics of Analytics?
The basics of analytics include data visualization, predictive modeling, statistical analysis, customer segmentation, and market trends. Techniques like decision trees, data mining, regression analysis, performance metrics, and correlation analysis drive insights and innovation.
What Is the Primary Focus of Business Analytics?
The primary focus of business analytics is to transform raw data into actionable insights through data interpretation, predictive modeling, and trend analysis. I leverage customer insights, performance metrics, and risk assessment to optimize processes and support strategic decision-making.
Conclusion
In wrapping up, business analytics isn't just a buzzword; it's a game-changer.
For instance, imagine a retail store predicting inventory needs using past sales data. By integrating descriptive, predictive, and prescriptive analytics, they'd streamline stock levels, reducing costs and maximizing profits.
This blend of data collection, statistical models, and actionable insights can transform decision-making in any industry.
So, embracing business analytics isn't optional; it's essential for staying competitive and informed.