When I implement AI predictive marketing, I start by using historical data to forecast customer behavior and trends. I guarantee data quality by gathering and preparing clean data from various sources. Choosing the right AI models aligned with marketing goals is vital. I then integrate these models into my marketing frameworks, utilizing data mining and social media insights. Measuring performance in real-time lets me track key metrics like conversion rates and ROI. Finally, I continuously optimize strategies to stay competitive and innovative. Want to elevate your marketing approach? Discover the methods that drive impactful results.
Key Takeaways
- Leverage historical data to predict customer behavior and personalize engagement strategies.
- Ensure high-quality, clean, and structured data for accurate predictive analytics.
- Select AI models that align with marketing goals and are scalable and interpretable.
- Integrate predictive models within marketing frameworks to optimize resource allocation and campaign performance.
- Continuously monitor performance metrics and adjust strategies based on real-time data insights.
Defining Predictive Analytics
Predictive analytics leverages historical data and patterns to forecast future marketing outcomes, transforming decision-making processes with precision and insight.
By implementing AI into marketing, I can analyze customer behavior, sales trends, and campaign performance effectively. This approach allows me to develop personalized strategies that enhance customer engagement and optimize campaigns.
Using predictive data analytics, I can anticipate customer churn, forecast sales trends, and automate personalized email campaigns. Tools like Pecan, Plat.AI, and DataRobot streamline this process by automating model building and data preprocessing, ensuring accurate predictions.
These strategies not only lead to more efficient resource allocation but also significantly enhance return on investment. Embracing AI-driven predictive analytics in marketing is essential for staying ahead in today's competitive landscape.
Data Collection and Preparation
Gathering and preparing high-quality data from various sources like CRM systems, social media platforms, and website analytics is imperative for generating accurate and actionable insights in AI predictive marketing. Effective data collection and data preparation guarantee the material we feed into predictive models is clean, organized, and structured. This foundation is crucial for reliable predictions, enabling informed decision-making.
High-quality data enhances customer segmentation and leads to optimized marketing strategies. By meticulously handling data, we derive accurate insights, which are pivotal for AI predictive marketing success. This diligent approach transforms raw information into powerful, actionable intelligence, driving innovation and achieving strategic marketing goals.
The better our data quality, the more effective and precise our predictions will be.
Choosing AI Models
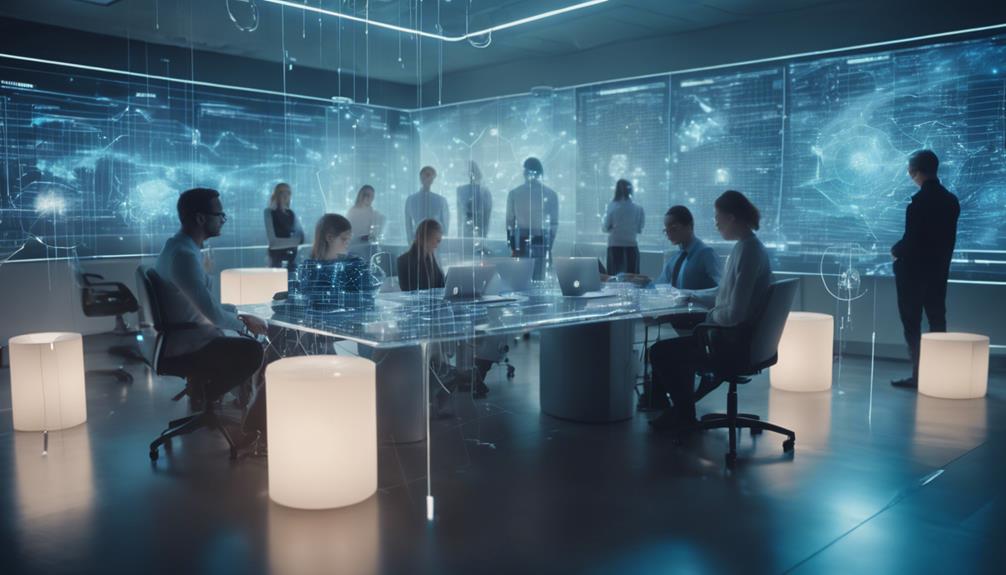
With our data meticulously prepared, it's time to strategically choose AI models that will transform this information into actionable marketing insights. When selecting AI models, I focus on aligning them with our specific marketing goals and the data available. I evaluate the complexity of the AI model to guarantee it meets our prediction accuracy needs. Scalability and interpretability are essential, as is proven success in similar marketing scenarios. Finally, I factor in the expertise and resources of our marketing team to make sure seamless implementation.
Here's a quick comparison to guide our selection:
Criteria | Considerations |
---|---|
Marketing Goals | Align with specific objectives |
Data Available | Suitability for data volume and variety |
Complexity | Necessary for accurate predictions |
Scalability | Ability to grow with our needs |
Interpretability | Ease of understanding and explaining results |
Proven Success | Track record in similar marketing contexts |
Integrating Predictive Models
When integrating predictive models, I focus on robust data collection techniques to guarantee high-quality inputs that underpin reliable predictions.
Deploying these models effectively requires strategic planning to seamlessly embed them within existing marketing frameworks.
Data Collection Techniques
Effective data collection techniques, such as data mining, surveys, social media listening, and customer feedback, are pivotal for integrating predictive models to uncover actionable insights. By utilizing these methods, I guarantee that my AI predictive marketing strategies are grounded in high-quality data.
Accurate data collection is the cornerstone for making precise forecasts and recommendations. Here's how I approach it:
- Data Mining: Extracting large datasets to identify patterns and trends.
- Surveys: Collecting structured responses directly from customers to gauge sentiment.
- Social Media Listening: Monitoring online conversations to capture real-time consumer opinions.
These techniques enable me to build robust predictive models that drive innovation and optimize marketing efforts, ensuring that the insights derived are both actionable and reliable.
Model Deployment Strategies
Integrating predictive models into marketing strategies transforms raw data into actionable insights, driving precise and timely decision-making. My approach to model deployment strategies starts with aligning predictive models to marketing objectives and KPIs. This alignment is vital for automating decision-making processes and optimizing marketing campaigns effectively.
Strategy | Benefits | Outcome |
---|---|---|
Aligning predictive models | Targeted marketing objectives | Increased ROI |
Automating decision-making | Streamlined processes | Real-time insights |
Customer segmentation | Improved audience targeting | Personalized marketing efforts |
Optimizing campaigns | Efficient resource allocation | Enhanced campaign performance |
Measuring Performance
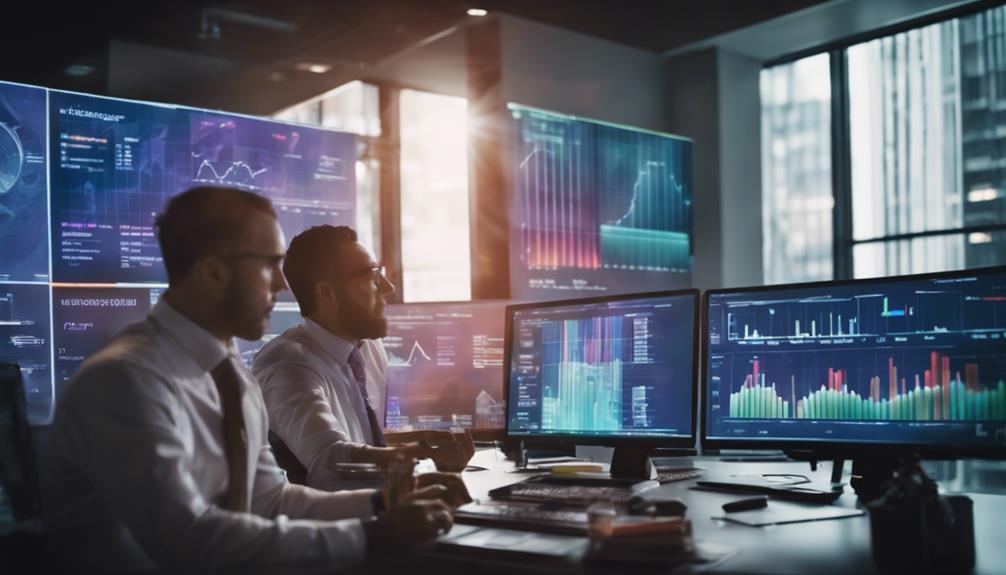
Tracking key metrics like conversion rates, customer retention, and ROI is crucial for gauging the success of AI predictive marketing campaigns. By leveraging AI tools, I can gain real-time insights into campaign performance, allowing me to optimize strategies effectively.
Performance measurement through AI analytics helps me identify successful tactics and areas needing improvement. This data-driven decision-making enhances marketing effectiveness and guarantees my efforts yield maximum impact.
Key metrics to monitor include:
- Conversion rates: Track how well my campaigns turn prospects into customers.
- Customer retention: Measure the loyalty and repeat business generated.
- ROI: Evaluate the financial returns of my marketing investments.
Incorporating these metrics with AI predictive marketing allows me to continuously refine my approach for superior results.
Continuous Optimization
When I focus on continuous optimization, I rely heavily on data-driven decision making to refine our marketing strategies in real-time.
By consistently monitoring performance metrics, I can adjust campaigns promptly to guarantee they align with our goals and maximize ROI.
This approach not only enhances campaign effectiveness but also keeps us adaptive to market changes and ahead of competitors.
Data-Driven Decision Making
Analyzing historical data to forecast future trends and customer behavior is essential for making informed, strategic decisions in AI predictive marketing. By leveraging data-driven decision making, I can enhance campaign performance and customer engagement through continuous optimization. This approach allows for agile adjustments to maximize ROI and drive customer satisfaction.
Key benefits include:
- Informed Decisions: Using historical data to predict customer behavior enables precise, timely marketing actions.
- Agile Adjustments: Continuous optimization guarantees strategies are adaptable, responding swiftly to new insights.
- Maximized ROI: Data-driven insights help allocate resources effectively, enhancing overall campaign efficiency.
Real-Time Performance Monitoring
Building on the foundation of data-driven decision making, real-time performance monitoring empowers marketers to make instantaneous adjustments that keep campaigns agile and effective. By leveraging live data, I can continuously optimize strategies to adapt to changing trends and customer behaviors.
AI algorithms play an essential role, analyzing real-time data to fine-tune ad targeting, content delivery, and customer engagement. This dynamic approach guarantees that my campaigns remain relevant and impactful in a constantly evolving digital landscape.
With real-time performance monitoring, I can make data-driven decisions on the fly, optimizing campaign performance and maximizing ROI. Continuous optimization isn't just a strategy; it's a necessity for staying competitive and innovative in today's fast-paced market environment.
Frequently Asked Questions
How to Maximize AI for Marketing?
I maximize AI for marketing by leveraging data analytics for customer segmentation, predictive modeling, and sales forecasting. Using consumer insights, I enhance personalization tactics, optimize campaigns, target behaviorally, and stay ahead of market trends to boost conversion rates.
What Is the Prediction of AI in Marketing?
Imagine predicting a customer's next purchase before they even think about it. AI's future in marketing hinges on predictive analytics, uncovering data patterns for market forecasting, demand prediction, and sales forecasting, revolutionizing consumer behavior insights and trend analysis.
How Artificial Intelligence Can Be Implemented in Marketing Strategies?
I can implement AI in marketing strategies by leveraging predictive analytics, customer segmentation, sentiment analysis, data mining, and churn prediction. This enables ad optimization, sales forecasting, trend analysis, campaign targeting, and a powerful personalization engine for innovative results.
How to Use AI in Marketing Automation?
Imagine harnessing AI to dive deep into customer segmentation and data analysis. I optimize campaigns, score leads, forecast trends, and predict churn using sentiment analysis. Behavioral targeting, content personalization, and multichannel integration drive my marketing automation success.
Conclusion
In predictive marketing, the journey doesn't end once the models are integrated. Remarkably, businesses using predictive analytics are 2.9 times more likely to report revenue growth. This stat underscores the importance of continuous optimization and performance measurement.
By consistently refining data collection and AI models, we can stay ahead of market trends and consumer behavior. Let's leverage these insights to drive our strategies and guarantee sustained success in a competitive landscape.