In real-time social sentiment analysis, I constantly monitor and interpret social media content using AI and machine learning. This process offers actionable insights by tracking brand perception, detecting trends, and spotting potential crises early. Key components include NLP for decoding language, machine learning for trend prediction, and real-time analysis for immediate feedback response. Though it faces challenges like unstructured and noisy data, advanced preprocessing and context-aware algorithms enhance accuracy. Applying these insights helps adjust marketing strategies on-the-fly, ensuring agility and relevance. If you're interested, you can explore how to leverage these tools for best decision-making.
Key Takeaways
- Real-time sentiment analysis uses AI to monitor and interpret social media content continuously.
- It provides immediate insights for quick strategy adjustments and operational efficiency.
- Advanced NLP and machine learning techniques decode language subtleties and detect sentiment intensity.
- Allows early detection of emerging trends and potential crises for proactive responses.
- Enhances marketing strategies by tailoring campaigns based on real-time public perception and feedback.
What Is Real-Time Social Sentiment Analysis?
Real-time social sentiment analysis involves continually monitoring and interpreting social media content to provide immediate insights into public opinions and emotions. By leveraging AI and machine learning, I can analyze vast amounts of social media data instantaneously. This allows me to discern public reactions and emotions towards brands or events in real-time.
Monitoring and analyzing such data enables me to track brand perception continuously, offering a dynamic view of how sentiments shift. Through real-time social sentiment analysis, I can detect emerging trends and potential crises early, allowing for swift responses. This constant flow of actionable insights guarantees I'm always informed, engaged, and ready to adapt strategies based on the latest public opinions and emotional reactions.
Importance of Real-Time Sentiment Analysis
Leveraging real-time sentiment analysis, businesses can gain immediate insights into public perception on social media platforms, enabling them to address issues and capitalize on positive trends swiftly. This proactive approach guarantees quick decision-making and timely responses. By monitoring sentiments in real-time, companies can fine-tune their strategies and maintain a competitive edge.
Here's a quick breakdown:
Benefit | Description | Outcome |
---|---|---|
Immediate Insights | Up-to-the-minute data on public perception | Informed strategy adjustments |
Proactive Issue Addressing | Early detection and resolution of problems | Enhanced customer satisfaction |
Capitalizing on Trends | Quickly leveraging positive feedback | Increased engagement |
Quick Decision-Making | Data-driven, real-time decisions | Operational efficiency |
Utilizing these tools effectively helps manage online reputation and stay ahead in a dynamic market.
Key Components of Sentiment Analysis Tools
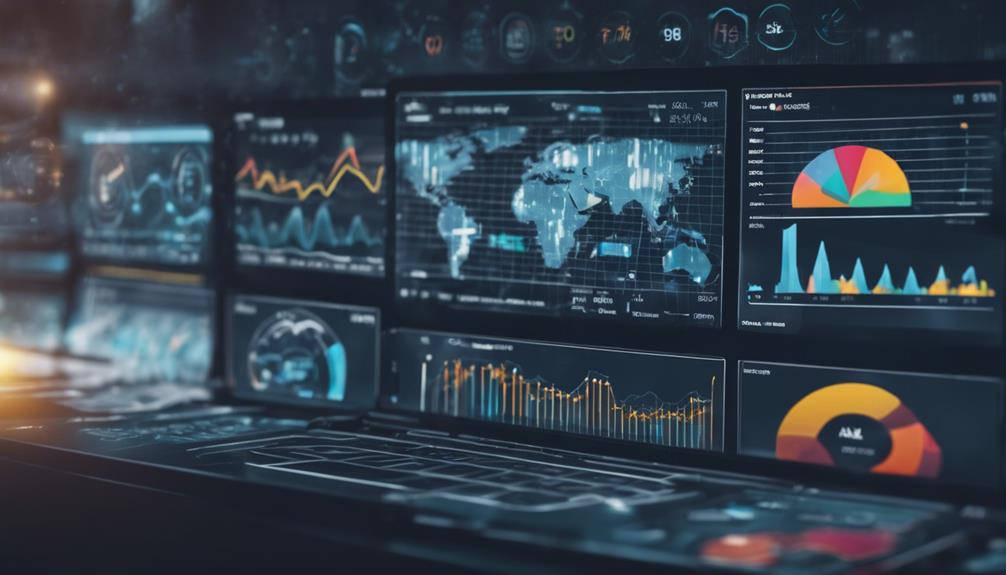
Analyzing sentiments effectively requires several key components in sentiment analysis tools. These components include natural language processing capabilities, machine learning algorithms, and robust data integration. Sentiment analysis tools leverage these elements to enhance Social Media Sentiment Analysis and improve Customer Experience.
Key components include:
- Natural Language Processing (NLP): Essential for decoding the subtleties of human language.
- Machine Learning Algorithms: Vital for identifying patterns and predicting sentiment trends.
- Real-Time Analysis: Allows for immediate reaction to Customer Feedback and Brand Reputation issues.
- Emotional Tone Detection: Helps in understanding the intensity and nature of sentiments.
- Data-Driven Decisions: Empowers businesses to adapt strategies based on insights.
These tools are indispensable for making data-driven decisions, ensuring robust brand reputation, and optimizing customer satisfaction.
Challenges in Social Sentiment Analysis
Despite the advanced capabilities of sentiment analysis tools, accurately interpreting social media sentiment presents several significant challenges. Handling unstructured data and noisy data from diverse social media platforms complicates the process. Sarcasm detection and grasping sentiment nuances demand sophisticated machine learning algorithms and domain-specific models. Contextual understanding, such as interpreting emojis and analyzing context factors, adds further complexity. Staying updated on brand conversations and employing precise terms are essential for success.
Challenge | Description | Strategy |
---|---|---|
Unstructured Data | Diverse formats and structures | Advanced preprocessing techniques |
Noisy Data | Irrelevant or misleading information | Filtering and cleaning methods |
Sarcasm Detection | Identifying sarcastic tone | Specialized machine learning models |
Contextual Understanding | Interpreting context and emojis | Context-aware algorithms |
Sentiment Nuances | Grasping subtle emotional tones | Fine-tuned domain-specific models |
Accurate sentiment analysis requires addressing these multifaceted issues.
Steps to Conduct Sentiment Analysis
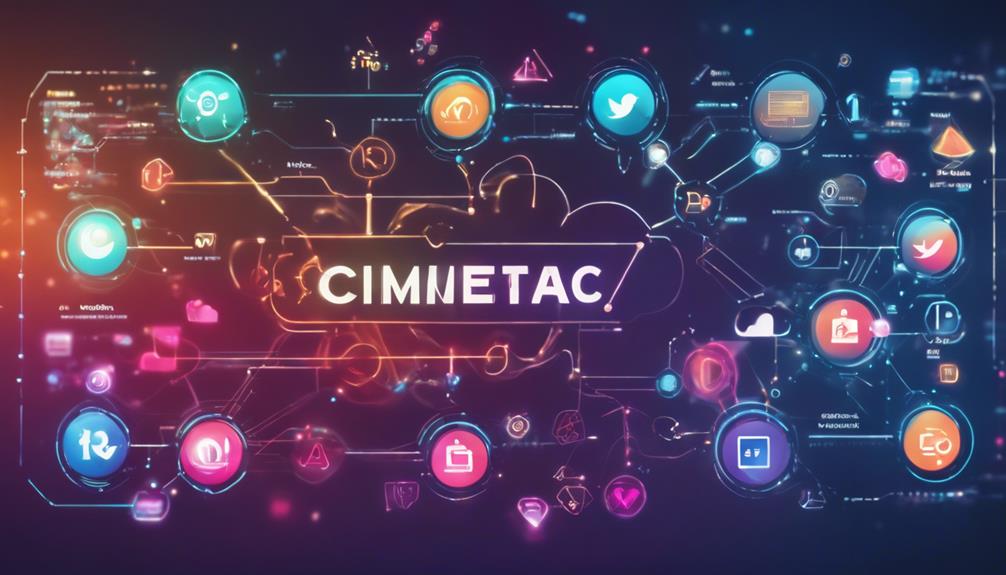
To conduct sentiment analysis effectively, I start by collecting data using social listening tools to gather a thorough dataset.
I then analyze the sentiment scores to identify trends, positive spikes, and areas of negative feedback.
These steps help me make informed decisions to address issues and enhance brand perception.
Data Collection Methods
When conducting sentiment analysis, we start by collecting text data from social media platforms like Twitter, Facebook, and Instagram using APIs, web scraping tools, or social media monitoring platforms. This process captures user-generated content such as posts, comments, and reviews.
Utilizing social media management tools like Sprinklr, Hootsuite, and Brandwatch, we can achieve real-time data collection to gain sentiment insights. This provides a thorough understanding of customer opinions and brand perception.
- APIs for direct data access
- Web scraping tools for broader data collection
- Social media monitoring platforms for real-time updates
- User-generated content for authentic insights
- Identifying emerging trends for proactive strategy
Analyzing Sentiment Scores
Conducting sentiment analysis involves a series of methodical steps, starting with data collection and preprocessing, followed by sentiment classification and feature extraction to accurately interpret sentiment scores. My sentiment analysis system uses sophisticated algorithms and machine learning models to analyze sentiments and assign sentiment scores ranging from -100 to 100. These scores help gauge customer sentiment and emotional tone, providing insights into the sentiment behind social sentiment data.
Step | Description |
---|---|
Data Collection | Gather sentiment data from various sources. |
Preprocessing | Clean and prepare data for analysis. |
Sentiment Classification | Classify text data into positive, negative, neutral. |
Feature Extraction | Extract key features influencing sentiment. |
Understanding sentiment scores allows businesses to track sentiment over time, enabling data-driven decision-making and strategy refinement.
Enhancing Sentiment Analysis With AI
I can enhance sentiment analysis with AI by employing machine learning algorithms and natural language processing to interpret complex emotions and linguistic subtleties.
Integrating real-time data guarantees that the analysis remains current and responsive to evolving trends.
This combination greatly boosts the accuracy and efficiency of sentiment analysis, allowing for more strategic decision-making.
Machine Learning Algorithms
Harnessing machine learning algorithms greatly enhances the precision and efficiency of real-time sentiment analysis in social data. By integrating natural language processing techniques, these algorithms enable automatic sentiment classification, efficiently managing vast amounts of unstructured social data.
Neural networks and deep learning models markedly improve the accuracy of sentiment detection, including complex nuances like sarcasm and irony. Supervised learning algorithms, trained on labeled datasets, classify sentiments as positive, negative, or neutral. Meanwhile, unsupervised learning identifies patterns and clusters in the data.
- Neural networks: Enhance detection of complex sentiments.
- Deep learning models: Improve accuracy and nuance recognition.
- Supervised learning: Trained on labeled data for precise classification.
- Unsupervised learning: Identifies hidden patterns in unstructured data.
Real-time analysis enables prompt sentiment classification and response.
Natural Language Processing
Natural Language Processing (NLP) supercharges sentiment analysis by enabling AI to accurately interpret and classify human emotions and context in real-time. NLP algorithms process text data, extracting nuanced sentiments and contexts, thereby enhancing sentiment analysis accuracy.
Techniques like tokenization and part-of-speech tagging dissect text into meaningful units, providing granular insights into emotions. Advanced NLP models like BERT and GPT-3 capture complex language nuances, making sentiment classification more precise.
AI-powered NLP tools can analyze vast amounts of text data swiftly, facilitating real-time social sentiment analysis. By leveraging NLP, we can transform raw text data into actionable sentiment insights, pushing the boundaries of what sentiment analysis can achieve in dynamic, real-world applications.
Real-Time Data Integration
Building on the capabilities of NLP, real-time data integration employs AI to process and analyze sentiment data as it's generated, enabling immediate and actionable insights. By leveraging AI technologies like machine learning and natural language processing, we can respond promptly to customer feedback and market trends. This integration delivers:
- Valuable insights into public perception and brand reputation.
- Enhanced speed and accuracy in sentiment analysis.
- Real-time monitoring of social channels.
- Immediate identification of emerging issues.
- Data-driven decision-making capabilities.
Real-time data integration guarantees businesses stay ahead by providing a constant flow of actionable data.
This not only fortifies brand reputation but also empowers us to adapt swiftly to dynamic market conditions.
Applying Insights to Marketing Strategies
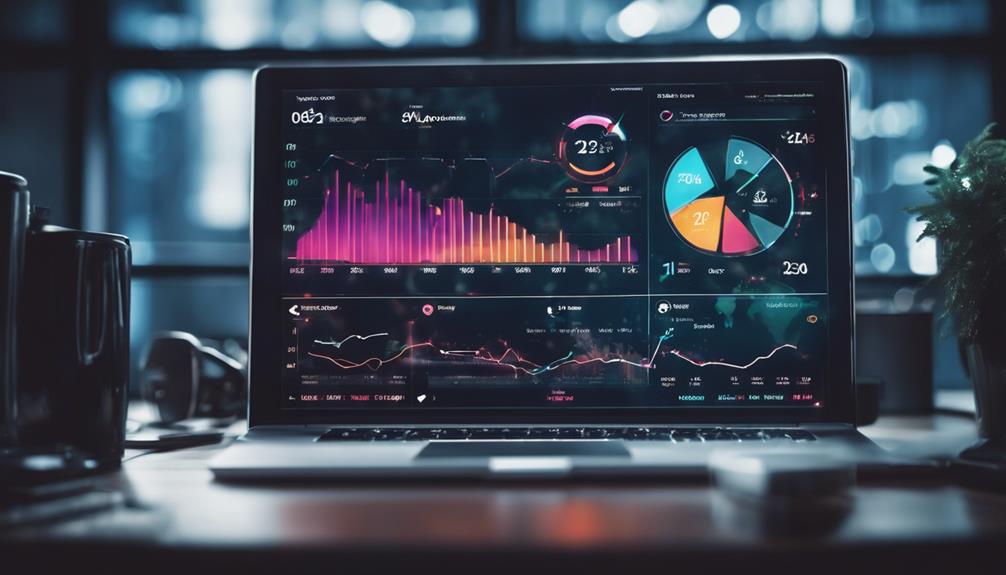
By leveraging real-time social sentiment analysis, we can promptly adjust our marketing strategies to align with evolving customer expectations and market trends. By utilizing immediate insights into customer perceptions, we tailor campaigns to resonate more effectively.
Real-time analysis allows us to address issues as they arise, guaranteeing our brand remains responsive and relevant. We capitalize on opportunities by identifying emerging trends early on, enhancing our targeted marketing efforts.
Immediate feedback guides us to adapt strategies on the fly, optimizing our approach for better engagement and conversion rates. Leveraging social sentiment analysis in real-time transforms our ability to respond dynamically, making our marketing strategies more agile and customer-focused. We make certain our initiatives are always in tune with the market pulse.
Frequently Asked Questions
How Do You Do Real Time Sentiment Analysis?
To do real-time sentiment analysis, I use data preprocessing and stream processing. I integrate APIs for text mining, apply language models for feature extraction, and use machine learning for sentiment scoring. Real-time monitoring and sentiment visualization guarantee actionable insights.
How to Do Social Sentiment Analysis?
Think of social sentiment analysis as listening to a crowded room using sophisticated tools. I leverage Natural Language Processing and Machine Learning to perform Emotion Detection, Text Classification, and Opinion Mining, extracting insights through Feature Extraction and Sentiment Scores.
How to Analyze Sentiment Analysis?
To analyze sentiment analysis, I first preprocess text, perform emotion classification, and use data annotation. I rely on sentiment lexicons, opinion mining, context analysis, feature extraction, and machine learning algorithms, measuring with sentiment metrics in natural language.
What Is the Best Approach for Sentiment Analysis?
For the best sentiment analysis, I harness the power of machine learning. I start with data preprocessing and feature extraction, use sentiment lexicons, neural networks, and language models. Model training and text classification enable precise emotion detection and opinion mining.
Conclusion
To sum up, real-time social sentiment analysis is vital for modern marketing strategies.
Did you know that 72% of customers who've had a positive social media experience with a brand are likely to recommend it to others? This statistic highlights the power of sentiment analysis in shaping brand perception.
By leveraging AI, we can decode vast amounts of social data swiftly, turning insights into actionable strategies. Embrace these tools, and you'll stay ahead in an ever-evolving digital landscape.