When evaluating the best predictive analytics tools, I'd focus on integration capabilities, user interfaces, and scalability. Altair AI Studio excels with seamless cloud integration and a low-code environment. H2O Driverless AI offers automated feature engineering and model interpretability. IBM Watson Studio supports collaboration and popular programming languages. Microsoft Azure Machine Learning boasts scalable infrastructure and AI-driven insights. SAP Predictive Analytics provides interactive forecasts and mobile accessibility. Each tool has unique strengths, essential for aligning with specific enterprise needs. Understanding these features can help pinpoint the best solution for sophisticated data analysis and forecasting.
Key Takeaways
- Altair AI Studio: Empowers real-time data access and advanced analytics in a collaborative, low-code environment.
- H2O Driverless AI: Offers automated feature engineering, hyperparameter optimization, and model interpretability for accessible and high-performance predictive modeling.
- IBM Watson Studio: Facilitates collaboration with integrated AI, machine learning, and seamless IBM Cloud integration for innovative analytics projects.
- Microsoft Azure Machine Learning: Provides scalable infrastructure and user-friendly interface, enhancing predictive analytics with AI-driven insights.
- SAP Predictive Analytics: Delivers interactive forecasts via generative AI, robust models, and mobile accessibility for enterprise-tailored predictive insights.
Overview of Predictive Analytics
Predictive analytics leverages advanced statistical techniques and data mining to forecast future trends and identify patterns from vast datasets.
Utilizing predictive analytics tools, I can connect data from multiple sources and employ machine learning algorithms to build robust predictive models. These tools integrate seamlessly with existing software platforms, enhancing our business capabilities by automating data analysis and delivering actionable insights.
By harnessing the power of predictive analytics, I can efficiently process large datasets, uncover hidden trends, and support strategic decision-making. The ability to forecast outcomes and detect patterns is essential for maintaining competitiveness and driving innovation in industries such as healthcare, finance, marketing, and manufacturing.
Predictive analytics is indispensable for shaping the future of business.
Key Features to Look For
When evaluating predictive analytics tools, prioritize features like integrated machine learning capabilities, diverse data sourcing options, and standalone utility to guarantee thorough data analysis and actionable insights.
Key predictive analytics features include robust AI integration, which empowers users to build sophisticated models. Tools should offer cloud-based solutions for scalability and flexible data access.
Effective data visualization and business intelligence capabilities are essential for interpreting complex datasets. Additionally, features for data preparation, such as cleaning and organizing data, are essential for accurate predictions.
A holistic tool should support varied data inputs, ensuring a complete view. By focusing on these features, users can harness the full potential of predictive analytics, driving innovation and informed decision-making.
Altair AI Studio

When evaluating Altair AI Studio, I find its key features, integration capabilities, and user experience particularly compelling.
The tool's advanced machine learning functions and intuitive interface stand out, making it accessible to both data experts and business users.
Additionally, its automation features greatly streamline the predictive modeling process.
Key Features Overview
Altair AI Studio integrates seamlessly with cloud data sources, offering a low-code interface that simplifies complex operations and enhances collaboration. This platform stands out in the predictive analytics landscape, providing essential tools for data science and machine learning.
Users can leverage advanced analytics and AI capabilities to build robust forecasting models and perform intricate data mining tasks. The platform's automated ML features streamline model creation, while data visualization tools aid in presenting insights effectively.
Key features include:
- Seamless cloud integration for real-time data access.
- Low-code environment for intuitive, user-friendly operations.
- AI-assisted insights enhancing decision intelligence.
- Advanced analytics empowering thorough data analysis.
Altair AI Studio's collaborative setting guarantees that teams can efficiently tackle complex predictive analytics projects.
Integration Capabilities
With seamless integration capabilities, Altair AI Studio guarantees users can effortlessly connect to various cloud data sources for enhanced data accessibility.
This integration fosters collaboration and sharing of predictive analytics models across diverse data ecosystems.
By accessing real-time data, users can derive accurate predictions and actionable insights.
Altair AI Studio's integration features promote a streamlined workflow, reducing time-to-insight and enhancing decision-making processes.
The platform's ability to integrate with cloud data sources empowers users to operate efficiently, maintaining a continuous flow of data for ongoing analysis.
User Experience Evaluation
Leveraging its robust integration capabilities, Altair AI Studio delivers a user-friendly experience that combines advanced data science and machine learning features for both experts and business users. The platform enhances accessibility, making it easy to navigate and utilize advanced analytics tools.
Here are key points highlighting its strengths:
- Accessible: Designed for both data science experts and business users, ensuring everyone can harness its power.
- Advanced Analytics: Offers extensive tools for developing predictive models, solidifying its place among top predictive analytics tools.
- Seamless Experience: Intuitive interface simplifies the process of analyzing data and deploying machine learning models.
- Robust Features: Integrates advanced machine learning capabilities, making complex tasks manageable.
Altair AI Studio stands out in 2024 for its innovative approach to predictive analytics tools.
H2O Driverless AI
When I think about H2O Driverless AI, its automated machine learning stands out, streamlining model creation with minimal manual input. Its model interpretability features offer transparency, helping users understand predictions and underlying data patterns.
This combination guarantees both efficiency and clarity in predictive analytics.
Automated Machine Learning
H2O Driverless AI revolutionizes automated machine learning by streamlining feature engineering, model selection, and hyperparameter optimization into a single, efficient workflow. This platform accelerates predictive analytics by automating complex tasks, making it ideal for both beginners and experts. Its advanced algorithms handle various data types and scale efficiently, ensuring accurate predictions and high interpretability.
Here's why H2O Driverless AI stands out:
- Automated Feature Engineering: Saves time by automating the creation of relevant features.
- Model Selection: Chooses the best model based on data characteristics and performance metrics.
- Hyperparameter Optimization: Fine-tunes model parameters for peak performance.
- Ease of Use: Offers a user-friendly interface suitable for all skill levels.
H2O Driverless AI truly transforms the landscape of predictive analytics.
Model Interpretability Features
Building on the efficiency of automated machine learning, Driverless AI also prioritizes model interpretability, offering robust tools to understand and trust the predictions made. By incorporating model interpretability features, it guarantees transparency and enhances trust in predictive models. Users can leverage feature importance metrics to identify key drivers of model decisions, while partial dependence plots provide insights into the relationships between features and predictions.
Additionally, SHAP values offer a granular view of each feature's contribution to the model's output. This transparency is essential for interpreting complex machine learning models, aiding in better decision-making. By providing these insights, H2O Driverless AI empowers users to demystify the inner workings of their predictive models, fostering more informed and confident decisions.
IBM Watson Studio
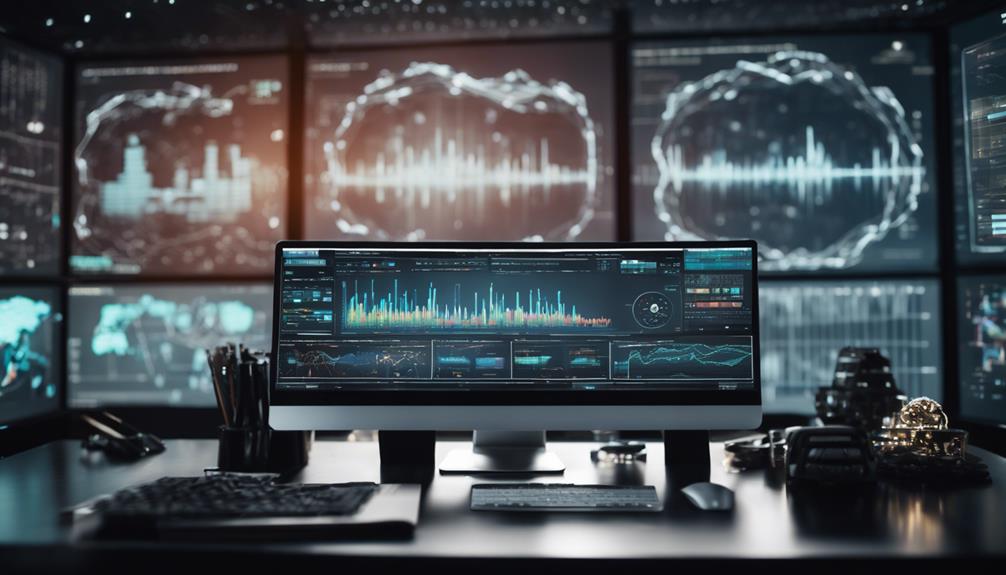
Leveraging its robust suite of tools for data preparation, model development, and deployment, IBM Watson Studio empowers data professionals to seamlessly collaborate on advanced analytics projects.
Data scientists can harness the power of predictive analytics using popular languages like Python and R programming. With integrated AI and machine learning capabilities, the platform enables sophisticated model building and analysis.
IBM Watson Studio's seamless integration with IBM Cloud services provides scalable computing resources, making it ideal for large datasets and complex algorithms.
Key features include:
- Data Preparation: Efficiently clean and organize data.
- Model Development: Utilize a wide range of algorithms for precise predictions.
- Deployment: Easily deploy models to production environments.
- Collaboration: Foster teamwork between data scientists, developers, and analysts.
This makes IBM Watson Studio a formidable tool for innovative data-driven decision-making.
Microsoft Azure Machine Learning
Microsoft Azure Machine Learning stands out for its scalable infrastructure and user-friendly tools, enabling efficient and effective machine learning model development. With a rating of 4.3 from 52 reviews, Azure Machine offers a robust set of features that make the machine learning process streamlined and accessible.
Its scalable infrastructure supports intensive machine learning model building, providing AI-driven insights that enhance predictive analytics capabilities. The platform's user-friendly interface simplifies complex tasks, making it easier for data scientists to generate precise models efficiently.
Microsoft's focus on integrating powerful, yet accessible, tools within Azure Machine Learning guarantees it remains a top choice for those looking to leverage predictive analytics software for innovation and advanced data solutions.
SAP Predictive Analytics
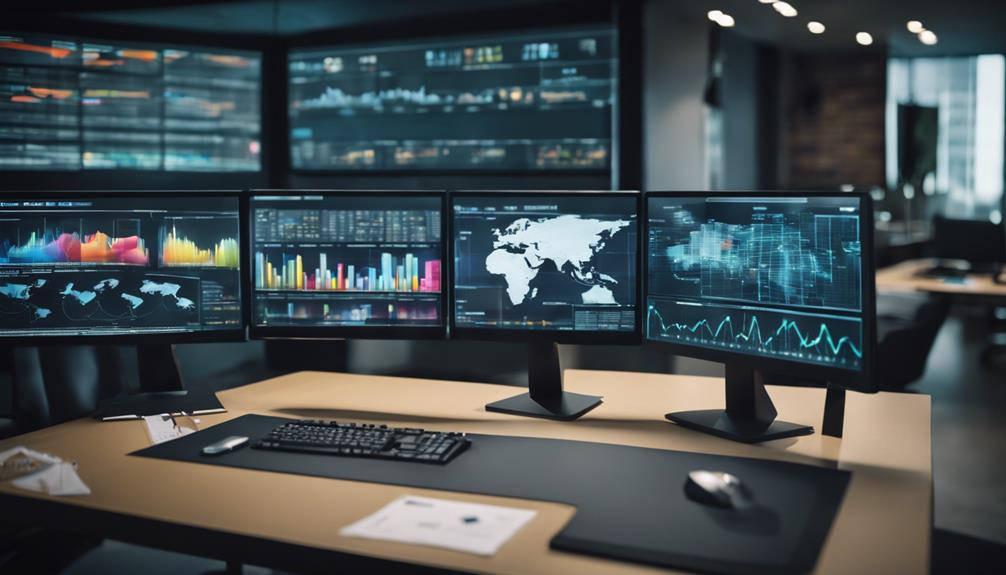
While Microsoft Azure Machine Learning excels in scalable infrastructure and user-friendly tools, SAP Predictive Analytics distinguishes itself with generative AI capabilities that create interactive forecasts and diverse data sourcing options for extensive analytics.
I particularly appreciate its user-friendly interfaces and collaborative features, which streamline workflow and enhance team productivity. The platform's simulations offer robust predictive models, essential for making data-driven decisions. Mobile accessibility guarantees that critical insights are always within reach, regardless of location.
Here are four key aspects:
- Generative AI: Enables creation of interactive forecasts.
- Diverse Data Sourcing: Facilitates in-depth analytics.
- Simulations: Provides robust predictive models.
- Mobile Accessibility: Ensures insights on-the-go.
Pricing information is customized, catering to specific enterprise needs, making it a versatile choice.
Choosing the Right Tool
Choosing the right predictive analytics tool starts with a clear understanding of your enterprise's specific needs and use cases.
First, evaluate existing tools like BI or CRM systems to determine if they meet your requirements or if new data science or industry-specific tools are necessary.
It's important to assess whether generic tools or those tailored to your industry provide the best fit.
Advancements in no-code AutoML tools offer simplified processes, making predictive analytics accessible to both experts and business users.
Examine the capabilities of each predictive analytics tool to make sure they align with your enterprise needs.
Frequently Asked Questions
Which Is the Best Tool for Predictive Analysis?
I believe the best tool for predictive analysis excels in data visualization, machine learning, R programming, and Python libraries. It should also guarantee forecast accuracy, robust model validation, handle time series, big data, ensemble methods, and neural networks.
Which Statistical Tool Is Most Useful in Predictive Analytics?
You won't believe it, but the most useful statistical tool in predictive analytics is a blend of regression models, decision trees, and neural networks. Combining linear regression, random forests, and logistic regression creates unparalleled forecasting accuracy and insight.
What Are the 4 Predictive Analytics?
The four predictive analytics are machine learning, data mining, predictive modeling, and business forecasting. They involve algorithm selection, data preprocessing, model validation, predictive maintenance, risk assessment, and pattern recognition to drive innovative decision-making.
What Is the Most Used Technique in Predictive Analytics?
Machine learning is the most used technique in predictive analytics. I leverage data mining, regression analysis, decision trees, neural networks, clustering algorithms, and time series for predictive modeling, ensuring accurate model evaluation and feature selection.
Conclusion
It's no coincidence that the best predictive analytics tools share key features like scalability, user-friendly interfaces, and robust data processing capabilities. Choosing the right tool hinges on your specific needs and resources.
By aligning those needs with the tool's strengths, you'll unleash the full potential of your data, driving smarter decisions and transformative outcomes.