By adopting machine learning tools, I can greatly reduce costs by transforming raw data into actionable insights. Using ML algorithms, I optimize pricing strategies, enhance inventory management, and streamline operations based on predictive analysis. Machine learning also boosts supply chain efficiency through accurate demand forecasting and proactive maintenance scheduling, reducing downtime and expenses. With precise data-driven recommendations, I make faster, informed decisions that drive operational excellence. Continuous monitoring and automation guarantee my processes are efficient and waste-free. Want to uncover the full potential of machine learning in cost savings? Keep going.
Key Takeaways
- Predictive Analytics: Anticipate demand and streamline operations to reduce costs and increase efficiency.
- Inventory Management: Optimize stock levels to minimize waste and enhance inventory accuracy.
- Pricing Strategies: Utilize ML algorithms to optimize pricing for increased profitability.
- Predictive Maintenance: Schedule maintenance proactively to reduce downtime and extend equipment lifespan.
- Automation: Automate routine tasks with AI chatbots to cut service costs and improve response times.
Understanding Machine Learning
Although machine learning might seem complex at first, it primarily involves training computers to analyze data, identify patterns, and make decisions without explicit programming.
By leveraging ML algorithms, I can perform sophisticated data analysis to uncover valuable insights that drive cost reduction and cloud cost optimization. These insights enable informed decisions on resource allocation, ensuring that optimization tools are effectively utilized.
Through predictive analysis, machine learning allows me to anticipate demand and streamline operations, resulting in improved efficiency. Understanding machine learning basics is essential for harnessing its potential; it's not just about automation but also about refining processes to maximize cost savings.
Embracing ML algorithms transforms raw data into actionable strategies, promoting innovation and operational excellence.
Cost Optimization Strategies
To optimize costs effectively, I employ machine learning tools to analyze historical data and predict future trends, enabling precise and proactive decision-making.
By leveraging advanced machine learning algorithms for demand forecasting, I enhance supply chain optimization, resulting in significant cost savings.
Implementing these algorithms in pricing strategy allows me to optimize pricing models for maximum profitability.
In inventory management, machine learning minimizes waste and boosts cost efficiency.
Predictive maintenance ensures that machinery operates smoothly, reducing unexpected downtime and associated costs.
Continuous monitoring of these cost-saving measures guarantees ongoing optimization.
Through these strategies, I achieve a robust framework for maximizing costs, ensuring my business remains competitive and innovative in a dynamic market.
Streamlining Processes
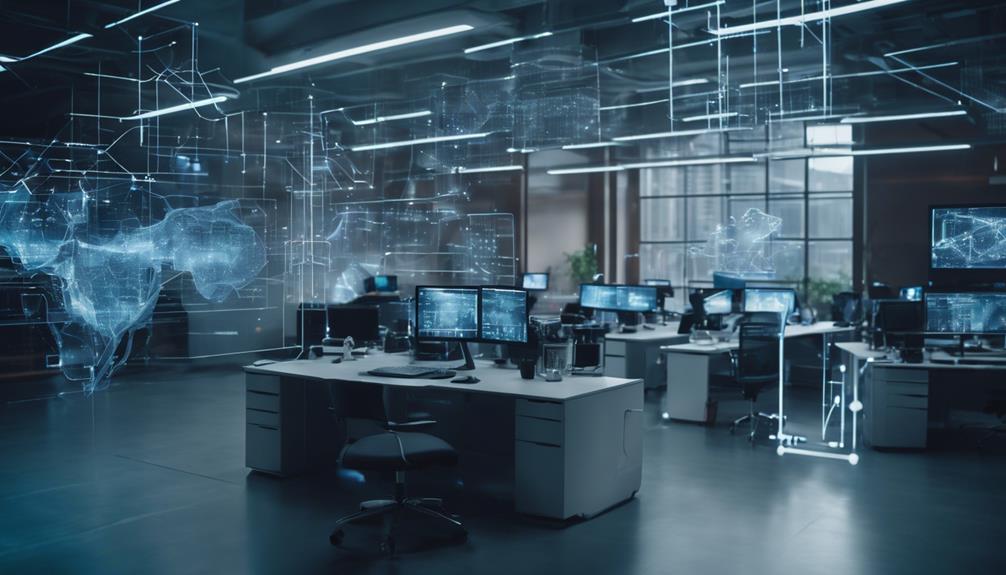
By implementing machine learning tools, I can automate complex tasks, greatly enhancing process efficiency and reducing operational costs. These tools streamline processes by automating data analysis, inventory management, and demand forecasting. By reducing human error, they improve operational efficiency and save valuable time.
Machine learning algorithms analyze data patterns, make accurate predictions, and optimize processes to maximize cost savings. This allows me to identify opportunities for further cost reduction and enhance decision-making for operational optimization. As a result, productivity increases, decisions are made faster, and resource allocation is improved.
The integration of machine learning tools in business operations is essential for achieving significant cost savings and maintaining a competitive edge in the market.
Enhancing Decision-Making
Machine learning tools consistently enhance decision-making by providing precise insights and data-driven recommendations. Analyzing historical data, these tools detect patterns and predict outcomes, optimizing decision-making processes.
By leveraging machine learning algorithms, I can identify cost-saving opportunities and streamline operations, thereby achieving significant cost savings and operational efficiency. This precision in decision-making not only reduces costs but also provides competitive advantages in our data-driven economy.
Automating complex tasks through machine learning allows for faster, more accurate decisions, essential for staying ahead in a competitive landscape. Ultimately, integrating machine learning into decision-making processes transforms how I manage resources, uncovering efficiencies that traditional methods often miss, driving sustainable growth and profitability.
Minimizing Waste
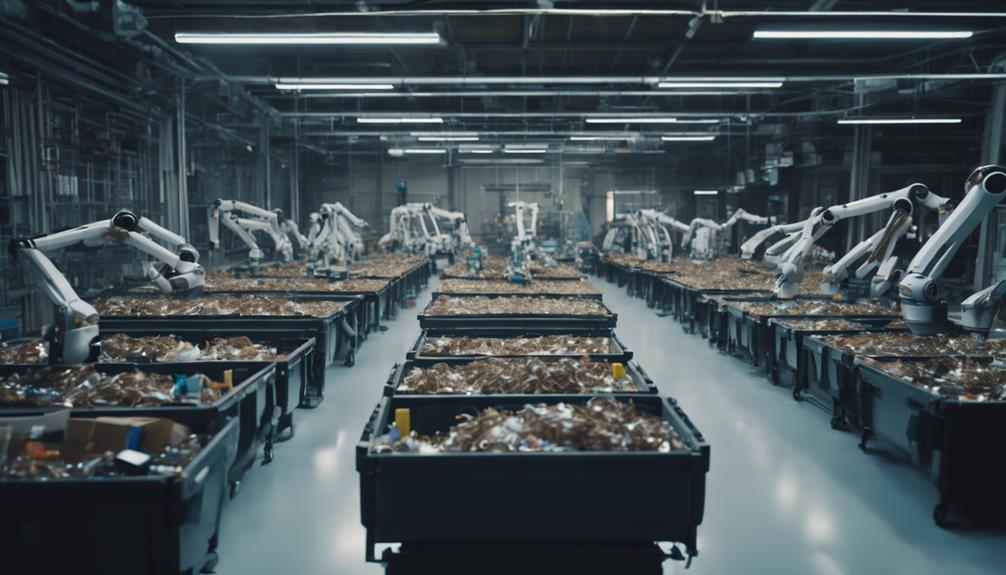
When it comes to minimizing waste, I utilize machine learning for predictive maintenance scheduling to prevent costly equipment breakdowns.
Inventory optimization techniques allow me to align stock levels with actual demand, reducing overstock and associated waste.
Additionally, efficient resource allocation through data-driven insights guarantees that every asset is utilized effectively, further cutting down on waste.
Predictive Maintenance Scheduling
Embracing predictive maintenance with ML guarantees heightened production efficiency and significant cost savings. Leveraging machine learning for predictive maintenance scheduling not only slashes maintenance costs by up to 30% but also cuts downtime by a striking 70%.
By analyzing equipment data with advanced ML algorithms, I can predict when maintenance is necessary, preventing breakdowns and minimizing waste. Machine learning tools empower me to optimize maintenance schedules, reducing unnecessary interventions and extending equipment lifespan.
Key benefits include:
- Reduced Maintenance Costs: Predictive maintenance lowers overall maintenance expenses.
- Improved Asset Utilization: Efficient scheduling enhances asset usage.
- Enhanced Production Efficiency: Minimizing downtime boosts production rates.
- Lower Repair Costs: Timely maintenance reduces the need for costly repairs.
Inventory Optimization Techniques
Building on the efficiency gains from predictive maintenance, I can enhance cost savings through inventory optimization techniques that minimize waste. By leveraging machine learning, I can accurately forecast demand, reducing excess inventory and preventing stockouts.
Predictive analytics allow me to analyze historical data, customer trends, and seasonality, optimizing inventory levels within the supply chain. Machine learning identifies slow-moving items, optimizes reorder points, and minimizes carrying costs.
Real-time monitoring and predictive modeling dynamically adjust inventory levels, greatly reducing waste. These machine learning algorithms improve inventory turnover rates and operational efficiency, contributing to substantial cost savings.
Efficient Resource Allocation
Machine learning tools enable us to allocate resources more efficiently by identifying usage patterns and optimizing utilization to minimize waste. By leveraging predictive analytics, we can anticipate future resource needs, preventing over-provisioning and enhancing Cloud Cost Management. Implementing machine learning models allows for:
- Efficient resource allocation: Continuously monitor and adjust resource utilization.
- Cost optimization: Identify potential cost-saving opportunities through precise data analysis.
- Proactive approach: Automate decisions to guarantee effective resource use.
- Anomaly detection: Spot irregularities in resource usage that may indicate inefficiencies.
Resource Allocation Optimization
By analyzing historical data and identifying usage patterns, we can optimize resource allocation to enhance cost efficiency and reduce waste. Machine learning tools play a pivotal role in this process by predicting future needs and automating decisions. This results in significant cost savings and improved operational efficiency. Leveraging real-time data, machine learning algorithms guarantee resources are distributed efficiently and cost-effectively.
Here's how it works:
Input Data | Process | Outcome |
---|---|---|
Historical Data | Analyze usage patterns | Predict future needs |
Usage Patterns | Develop optimization models | Optimize resource allocation |
Real-Time Data | Adjust allocations dynamically | Maximize cost savings and efficiency |
Real-World Success Stories
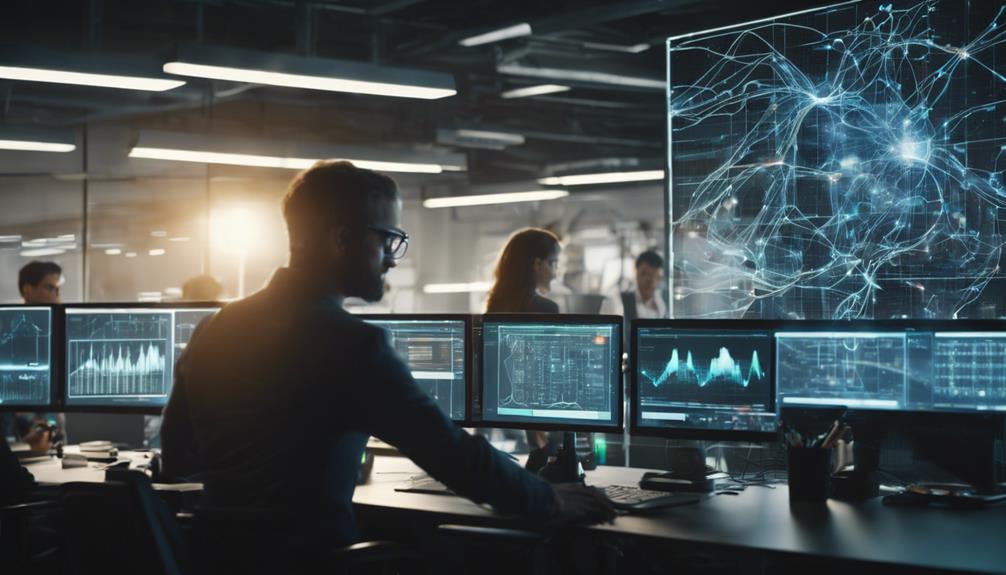
I've seen impressive results in retail inventory optimization and predictive maintenance implementation using machine learning. Retailers have cut costs by predicting demand more accurately, leading to better inventory management.
Similarly, predictive maintenance has minimized downtime and reduced expenses by forecasting equipment failures before they occur.
Retail Inventory Optimization
How have some of the largest retailers like Walmart and Amazon achieved significant cost savings through machine learning-driven inventory optimization? By leveraging advanced machine learning algorithms, these industry leaders have revolutionized inventory management.
Here's how:
- Walmart: Implemented machine learning for inventory optimization, saving $86 million annually through improved inventory accuracy and stock levels.
- Amazon: Reduced inventory costs by 20% by using machine learning tools to optimize retail inventory.
- Macy's: Increased inventory turnover by 8% with machine learning-driven demand forecasting and inventory optimization.
- Lowe's: Improved inventory accuracy by 98% through machine learning solutions, minimizing stock discrepancies.
These success stories highlight the transformative impact of machine learning in the retail industry, driving significant cost savings and operational efficiencies.
Predictive Maintenance Implementation
Leveraging machine learning for predictive maintenance has demonstrably reduced maintenance costs by 25% and decreased downtime by 70% for a global manufacturing company. By integrating ML algorithms, we've observed significant improvements in equipment efficiency and reliability. Real-world success stories reveal a 30% increase in equipment lifespan and a 20% boost in overall equipment efficiency. Additionally, unplanned downtime has been cut by 40%, and equipment availability has risen by 15%. These implementations have saved companies up to $1 million annually and reduced emergency maintenance incidents by 45%.
Metric | Improvement |
---|---|
Maintenance Costs | -25% |
Downtime | -70% |
Equipment Lifespan | +30% |
Equipment Efficiency | +20% |
Harnessing predictive maintenance is essential for those seeking to innovate and optimize operational efficiency.
Chatbots and AI Integration
Integrating AI-powered chatbots into customer support systems can greatly reduce operational costs while improving response times and customer satisfaction. By leveraging AI, businesses can automate up to 80% of routine queries, achieving a 30% savings in customer support costs.
AI-driven chatbots not only enhance operational efficiency but also reduce service costs by 33%. Moreover, personalized interactions provided by these chatbots lead to a 30% increase in customer satisfaction.
Here's how AI chatbots contribute to cost savings and efficiency:
- Automate routine interactions: Handle up to 80% of common queries.
- Enhance response times: Reduce response times by 99%.
- Improve operational efficiency: Achieve 24/7 support presence.
- Boost customer satisfaction: Increase satisfaction by 30%.
Integrating AI chatbots is a strategic move for any cost-conscious organization.
Future Trends
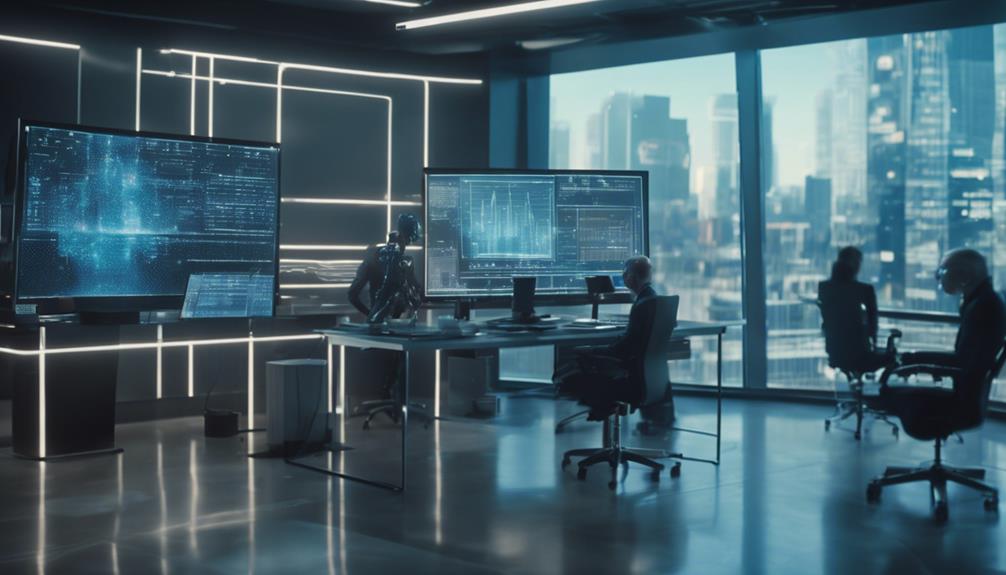
As we look ahead, machine learning tools promise not just to automate routine tasks but to fundamentally transform cost management through advanced predictive capabilities and anomaly detection. AI integration will drive proactive financial stewardship, allowing for precise resource allocation and autonomous cost management adjustments.
With advanced predictive analysis, businesses can forecast future resource needs accurately, optimizing costs efficiently. Anomaly detection will identify irregularities in cloud usage and expenses, ensuring that cost optimization strategies are continuously refined. Automation in ML will lead to strategic, real-time adjustments, enhancing overall financial management.
Embracing these trends will empower organizations to stay ahead of financial challenges, leveraging technology for smarter, more efficient cost savings.
Frequently Asked Questions
How Can Cost Optimization Be Achieved in Machine Learning Projects?
To achieve cost optimization in machine learning projects, I focus on data efficiency, model simplification, effective resource allocation, utilizing cloud infrastructure, automated tuning, predictive maintenance, strategic feature selection, scalable architectures, and rigorous algorithm benchmarking.
How to Use AI to Reduce Cost?
Juxtaposing efficiency with innovation, I use AI to reduce costs via predictive maintenance, optimizing supply chain, precise resource allocation, detecting fraud, cutting energy consumption, enhancing customer segmentation, minimizing waste, accurate demand forecasting, and refining inventory management.
What Are the Techniques Used in Machine Learning for Price Optimization?
For price optimization, I use dynamic pricing, demand forecasting, price elasticity, and customer segmentation. Revenue management leverages competitive pricing, personalized discounts, market analysis, and price simulation to boost profitability and customer satisfaction effectively.
What Is AI Driven Cost Optimization?
AI-driven cost optimization uses data analysis, predictive analytics, and workflow automation to enhance operational efficiency. It improves demand forecasting, resource allocation, and expense management, leading to significant cost reduction and better financial planning.
Conclusion
To wrap up, harnessing machine learning tools isn't just a game-changer, it's the bee's knees for cost savings. By optimizing resource allocation, streamlining processes, and enhancing decision-making, we've seen tangible benefits.
Real-world success stories and future trends highlight the transformative power of AI and chatbots. Let's continue embracing these innovations to make sure we're not just keeping up but staying ahead in a rapidly evolving landscape.
The future's bright, and the savings are substantial.