I've found that harnessing machine learning tools is essential for maximizing cost savings. By analyzing data, these tools help identify inefficiencies and streamline operations. Automated tasks and predictive maintenance reduce errors and downtime. AI-driven tools enhance decision-making, allowing for precise sales forecasting and dynamic pricing strategies. Implementing AI chatbots automates customer interactions, reducing the need for human agents and cutting costs. Future trends suggest even greater savings through advanced predictive analytics and resource optimization. To fully realize the potential of machine learning for cost savings, understanding these strategic implementations is imperative.
Key Takeaways
- Automate expense tracking to monitor and manage expenditures in real-time.
- Implement predictive maintenance to reduce equipment downtime and maintenance costs.
- Utilize AI-driven sales forecasting for precise inventory management and risk reduction.
- Deploy AI chatbots to automate customer interactions and minimize reliance on human agents.
- Leverage dynamic pricing strategies to optimize revenue and adapt to market demands.
The Impact of Machine Learning
How exactly does machine learning drive cost savings in businesses?
By leveraging machine learning tools, I can analyze vast amounts of data, identify patterns, and generate insights that drive strategic decision-making. Automated processes streamline operations, enhancing operational efficiency and reducing errors.
Predictive maintenance minimizes downtime and repair costs, while demand forecasting guarantees ideal inventory levels, avoiding overstock and stockouts. This data-driven approach leads to substantial expense reduction and operational efficiency.
Machine learning tools empower me to make informed decisions that improve profitability. By harnessing these technologies, I gain a competitive advantage, positioning my business to outperform competitors.
Implementing machine learning for cost savings isn't just about cutting costs; it's about creating a smarter, more agile business.
Cost Reduction Strategies
When I consider cost reduction strategies, I focus on automated expense tracking and predictive maintenance scheduling.
Using machine learning, I can automate the monitoring of expenditures and identify inefficiencies in real-time.
Additionally, predictive maintenance scheduling enables proactive management of equipment, reducing downtime and repair costs.
Automated Expense Tracking
Automated expense tracking leverages machine learning algorithms to greatly enhance cost reduction strategies by minimizing manual errors and optimizing budget allocations. By employing machine learning tools, I can streamline expense tracking, identify cost-saving opportunities, and automate budget allocations with precision.
Real-time insights allow me to proactively monitor expenditures and make data-driven decisions, ensuring peak cost management. Anomaly detection alerts me to unusual spending patterns, preventing potential overspending. Additionally, personalized recommendations help tailor cost reduction strategies to my specific needs.
This automated tracking not only improves accuracy but also saves up to 75% of the time traditionally spent on expense management tasks. By integrating these advanced tools, I can significantly optimize costs and achieve substantial financial efficiency in my operations.
Predictive Maintenance Scheduling
Building on the efficiencies gained through automated expense tracking, predictive maintenance scheduling with machine learning offers a robust strategy for further reducing operational costs and enhancing equipment reliability. By leveraging machine learning algorithms, I can analyze historical data to predict equipment failures, enabling proactive maintenance.
This approach leads to significant cost savings, reducing maintenance costs by up to 30% and increasing equipment uptime by 20%. ML-driven predictive maintenance optimizes maintenance schedules, minimizes operational disruptions, and extends asset lifespan by avoiding unplanned downtime.
In fact, such proactive strategies can reduce breakdowns by 70-75%, translating into fewer repair costs and maximized operational efficiency. This innovation is essential for businesses seeking long-term cost savings and reliability.
Enhancing Operational Efficiency
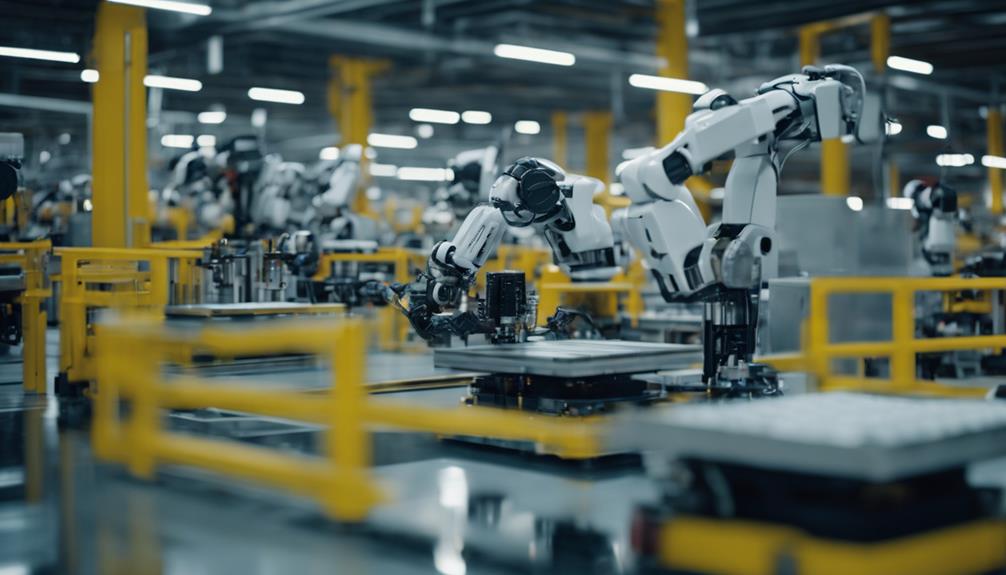
Machine learning tools greatly enhance operational efficiency by automating repetitive tasks and minimizing human errors. By implementing automation, we streamline operations, leading to substantial cost savings.
These tools analyze vast amounts of data, identifying patterns and making predictions that optimize processes. This not only improves decision-making but also uncovers cost-saving opportunities that were previously overlooked.
Enhanced productivity is achieved through the reduction of manual interventions, which in turn reduces operational costs. The predictive capabilities of machine learning further refine our operational strategies, ensuring maximum efficiency.
Ultimately, leveraging machine learning tools drives significant improvements in performance and overall business outcomes, firmly establishing them as essential for any forward-thinking organization aiming for innovation and efficiency.
Data-Driven Decision Making
Leveraging the predictive capabilities of machine learning, we can harness historical data to make informed, data-driven decisions that optimize costs and improve efficiency. By utilizing machine learning tools, I can analyze past performance to identify patterns and trends that reveal actionable insights.
This enables me to make strategic cost-saving choices and adapt swiftly to changing market conditions. Real-time data analysis further enhances my ability to monitor operations and make timely, informed decisions.
Automating these processes guarantees efficient operations, minimizing manual intervention and reducing errors. Ultimately, data-driven decision-making empowers me to optimize costs effectively, guaranteeing that every move is backed by precise, data-derived intelligence, leading to sustained cost-saving and operational excellence.
Real-World Applications
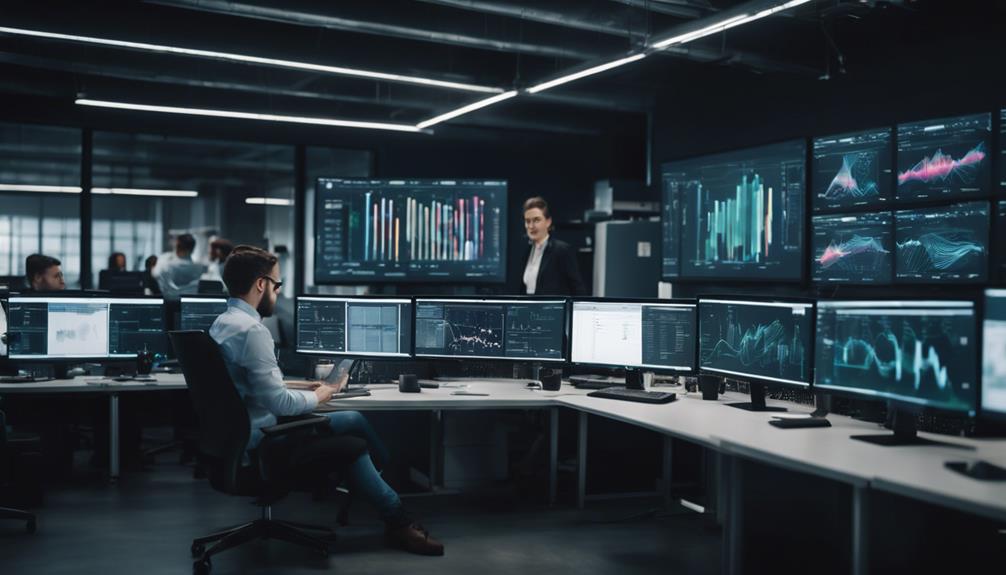
I'll now focus on key real-world applications of machine learning that drive cost savings.
Predictive maintenance enhances operational efficiency by anticipating equipment failures.
Inventory management optimization and dynamic pricing strategies further streamline costs and maximize profitability.
Predictive Maintenance Efficiency
Predictive maintenance, driven by machine learning algorithms, not only forecasts equipment failures with remarkable accuracy but also streamlines maintenance schedules to enhance operational efficiency. By analyzing historical data and sensor readings, machine learning tools can predict when equipment performance will decline, reducing unplanned downtime and maintenance costs.
Key Aspect | Benefit | Impact on Operations |
---|---|---|
Equipment Failures | Detected early | Prevents breakdowns |
Maintenance Costs | Reduced by up to 30% | Significant cost savings |
Maintenance Schedules | Optimized | Enhances operational efficiency |
Unplanned Downtime | Minimized by 70-75% | Increases equipment uptime |
These tools lead to significant cost savings by extending equipment lifespan and minimizing unnecessary maintenance. The efficiency gained from predictive maintenance is transformative, allowing companies to focus on innovation and growth.
Inventory Management Optimization
While predictive maintenance brings remarkable efficiency to equipment management, AI-driven inventory management tools like Inventoro offer equally transformative benefits for optimizing inventory levels and reducing operational costs.
By leveraging machine learning, these tools provide precise sales forecasting, ensuring inventory levels are optimized to meet demand without overstocking. This reduces costs and minimizes waste. Additionally, AI enhances decision-making insights, leading to better risk management and improved order fulfillment.
Real-world applications show that businesses utilizing AI for inventory management experience streamlined operations and reduced manual labor. The result is significant cost savings and improved operational efficiency.
Ultimately, AI-driven inventory optimization maximizes investments, enhances profitability, and prevents costly errors, making it indispensable for modern inventory management.
Dynamic Pricing Strategies
In leveraging machine learning algorithms, dynamic pricing strategies enable companies to adjust prices in real-time based on fluctuating market conditions and customer behavior.
By analyzing real-time data, these algorithms detect demand patterns and optimize prices, offering significant cost-saving opportunities.
For instance, Amazon and Uber use dynamic pricing strategies to maintain a competitive edge and maximize revenue.
Airlines adjust ticket prices based on seat availability and time to departure, while e-commerce platforms like eBay modify product prices to stay competitive.
Tools like PriceLabs for vacation rentals utilize machine learning to achieve price optimization amid varying market conditions.
Implementing a robust dynamic pricing strategy ensures competitive pricing and revenue optimization, directly enhancing a company's financial performance.
Chatbots and AI Integration
AI-powered chatbots automate customer interactions, significantly reducing the workload on human agents and driving cost savings. By integrating Machine Learning and AI, these chatbots enhance customer support through:
- Automation: Streamlining processes, improving operational efficiency, and enabling 24/7 support.
- Data-driven decision-making: Leveraging real-time data to optimize customer interactions and personalize support.
- Cost savings: Minimizing the need for human agents, reducing operational costs, and enhancing customer experience.
AI integration in chatbots allows for personalized customer support, responding instantly and improving responsiveness. This automation not only drives operational efficiency but also guarantees continuous service availability.
Data-driven decision-making further refines interactions, making them more effective and tailored to individual needs. Ultimately, AI-powered chatbots are pivotal in maximizing cost savings and enhancing overall customer satisfaction.
Future Trends in Cost Optimization

Building on the efficiency gains from AI-powered chatbots, future trends in cost optimization are set to leverage advanced AI and ML for enhanced predictive analytics and autonomous financial adjustments. Machine Learning will greatly elevate cost optimization through its predictive capabilities and autonomous management. AI solutions will enable precise forecasting and proactive financial stewardship, identifying cost-saving opportunities.
Businesses will align cloud spending with specific objectives, ensuring efficient resource utilization. By integrating AI and ML, companies can pinpoint irregularities in cloud usage and expenses, driving innovation and sustainability. As Forbes notes, nearly half of businesses foresee AI playing an essential role in future cost optimization initiatives, underscoring the importance of embracing these advanced technologies for competitive advantage.
Frequently Asked Questions
How Can Cost Optimization Be Achieved in Machine Learning Projects?
To achieve cost optimization in machine learning projects, I focus on algorithm efficiency, data preprocessing, model selection, feature engineering, hyperparameter tuning, batch processing, resource allocation, cloud services, performance monitoring, and version control to guarantee streamlined, cost-effective operations.
How to Use AI to Reduce Cost?
To reduce cost with AI, I leverage predictive maintenance to prevent equipment failures, optimize inventory management, use demand forecasting for accurate supply chain decisions, enhance energy efficiency, detect fraud, automate processes, implement dynamic pricing, and streamline workforce management.
What Are the Techniques Used in Machine Learning for Price Optimization?
Diving into dynamic pricing, I use clustering algorithms for customer segmentation, regression analysis for price elasticity, and demand forecasting to drive decisions. Predictive analytics and reinforcement learning refine strategies, while feature engineering enhances precision in price discrimination.
What Is AI Driven Cost Optimization?
AI-driven cost optimization leverages predictive analytics, demand forecasting, and dynamic pricing to enhance resource allocation and operational efficiency. It integrates data for anomaly detection, risk management, and decision support, optimizing supply chain processes for innovative solutions.
Conclusion
To conclude, machine learning isn't just a futuristic concept; it's a practical tool for maximizing cost savings now. While traditional methods can yield slow, inconsistent results, machine learning offers rapid, data-driven insights.
By juxtaposing the inefficiencies of past strategies with the precision of AI, it's clear: embracing these technologies isn't optional—it's essential. With chatbots and AI integration, operational efficiency skyrockets, and the future of cost optimization looks brighter than ever.