I optimize programmatic ad targeting with machine learning by analyzing vast datasets to pinpoint the ideal audience at the perfect time. Using AI, I predict user behavior and preferences for personalized ads, boosting conversion rates and ROI. Training models with labeled data enhances predictive analytics, while unsupervised learning reveals hidden audience patterns. Real-time data analysis identifies high-value segments and adapts swiftly to shifting user dynamics. Techniques like clustering and regression analysis refine audience segmentation for precise targeting. Predictive modeling transforms historical data into insights that maximize ad relevance and campaign performance. There's a lot more to uncover about these strategies.
Key Takeaways
- Machine learning algorithms analyze user behavior for precise audience targeting.
- Real-time data analysis optimizes ad placement for high engagement.
- Predictive modeling forecasts user interactions to enhance ad relevance.
- Audience segmentation techniques tailor ads for increased conversion rates.
- Reinforcement learning refines bid strategies for maximized ROI.
Understanding Programmatic Ad Targeting
When we explore programmatic ad targeting, we see how machine learning algorithms meticulously analyze vast datasets to pinpoint specific audience segments for more effective ad delivery. By leveraging AI in programmatic advertising, I can optimize ad placements in real-time, ensuring that the ads reach the right audience at the right moment.
This data analysis enhances ad performance by predicting user behavior and interests. Personalized ads become more relevant, driving higher engagement. Audience segmentation is essential here; it allows me to tailor ads precisely, increasing conversion rates and ROI.
The ability to deliver relevant ads based on user demographics, behavior, and context is what makes machine learning indispensable in programmatic ad targeting. It's the future of precise, effective advertising.
Machine Learning Algorithms
To fully harness the power of programmatic ad targeting, I rely on sophisticated machine learning algorithms that analyze and interpret vast datasets for the most effective ad placements. These algorithms enhance ad campaigns by:
- Training machine learning models with labeled data to improve Predictive Analytics and ad optimization.
- Utilizing unsupervised learning to uncover hidden patterns, enabling personalized ad strategies.
- Applying reinforcement learning to refine bid optimization and user engagement through continuous learning.
- Leveraging AI and Machine Learning to guarantee ads reach the right audience at the right time.
Real-Time Data Analysis
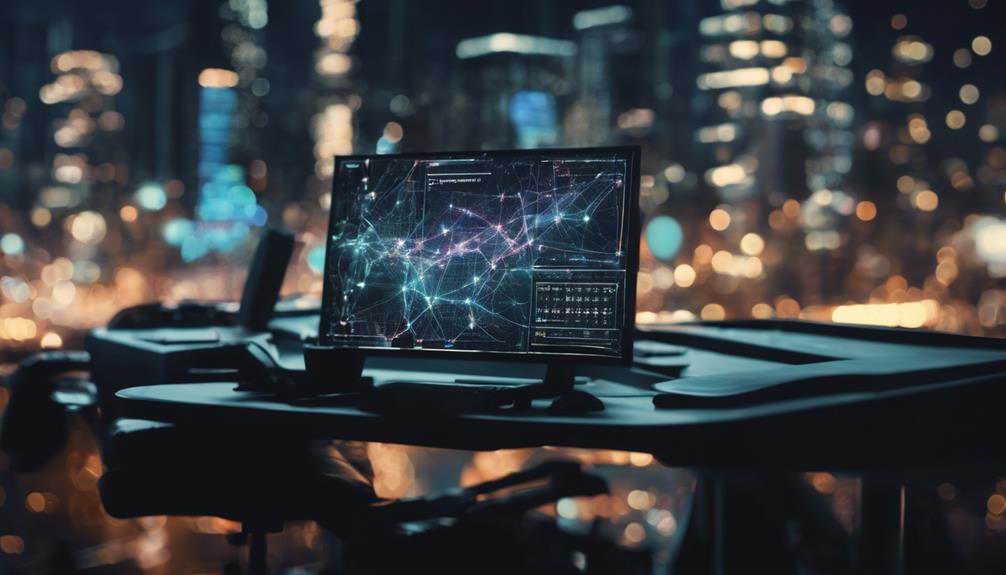
Harnessing real-time data analysis, I can instantly process vast datasets to make precise and immediate targeting decisions in programmatic advertising. Machine learning algorithms enable me to analyze user behavior, preferences, and contextual data in real time, allowing for dynamic adjustments to ad placements based on audience interactions.
This instant data processing identifies high-value audience segments and optimizes ad targeting by adjusting bids accordingly. By leveraging real-time data, I can enhance ad relevancy and improve campaign performance. This approach guarantees that my ads reach the right audience at the right time, maximizing ROI.
Real-time analysis is essential for staying ahead in the competitive landscape of programmatic ad targeting, providing the agility needed to adapt to ever-changing user dynamics.
Audience Segmentation Techniques
Leveraging the power of real-time data analysis, I employ advanced audience segmentation techniques to divide consumers into specific groups based on shared characteristics or behaviors. By harnessing machine learning in programmatic advertising, I optimize targeting strategies to deliver personalized ads.
Key techniques include:
- Clustering: Grouping users with similar behaviors to identify target segments.
- Regression Analysis: Predicting user responses and preferences to tailor ads.
- Decision Trees: Classifying audiences based on multiple criteria for precise targeting.
- Data Analysis: Continuously refining segments to enhance ad engagement.
These methods boost ad engagement and return on ad spend by ensuring ads reach the right audience.
Effective audience segmentation is integral to driving innovation in digital advertising.
Predictive Modeling Applications
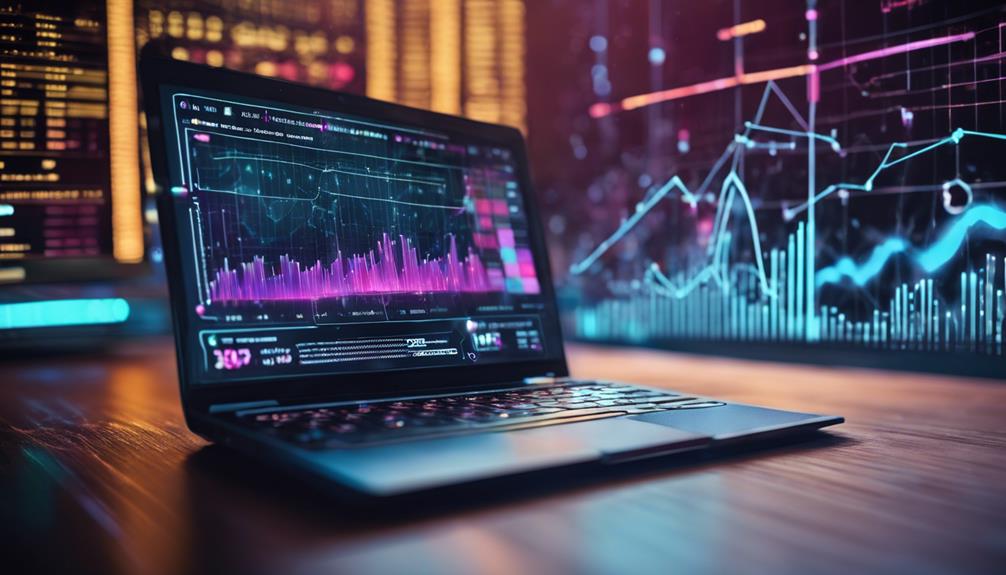
Predictive modeling transforms historical data into actionable insights, enabling me to anticipate user behaviors and optimize ad placements with precision. Leveraging machine learning, I can analyze user behavior patterns to forecast ad engagement.
This predictive modeling approach in programmatic ad targeting allows for more data-driven decisions, enhancing ad relevance and improving campaign performance. By targeting audiences more effectively, I can guarantee efficient ad spend and maximize conversions.
Predictive modeling applications help me allocate ad placements strategically, increasing the likelihood of reaching the right audience at the right time. Ultimately, this enables a more refined and impactful advertising strategy, driving significant improvements in ad performance and overall campaign outcomes.
Fraud Detection and Prevention
When tackling ad fraud, I focus on identifying anomalous patterns and real-time threat mitigation. Machine learning algorithms analyze vast datasets to spot irregularities like click fraud or bot traffic.
Identifying Anomalous Patterns
Machine learning algorithms excel at detecting anomalies in ad traffic data, playing a pivotal role in identifying and preventing ad fraud. By analyzing vast amounts of ad traffic data, machine learning models can pinpoint anomalous patterns that may signify fraudulent activities. These anomalies, such as sudden spikes in click-through rates or unusual user behavior, are flagged for further investigation.
Machine Learning:
Continuously adapts to evolving fraud tactics.
Ad Fraud Detection:
Identifies fraudulent activities in programmatic advertising.
Real-Time Adaptation:
Learns from data patterns to stay ahead of fraudsters.
Financial Losses:
Drastically reduced through proactive detection.
In an industry where financial losses due to ad fraud are substantial, leveraging machine learning for ad fraud detection ensures a robust defense against evolving fraud tactics.
Real-Time Threat Mitigation
Building on the ability to identify anomalous patterns, real-time threat mitigation leverages machine learning to analyze ad traffic instantaneously, guaranteeing immediate responses to potential fraud. By utilizing real-time data analysis, we can enhance ad fraud prevention in programmatic advertising.
Machine learning models excel at predicting fraudulent behavior by examining historical data analysis, allowing for more refined and accurate fraud detection. Automation in ad targeting enables swift threat response, drastically reducing fraud risk in programmatic ad campaigns.
This proactive approach not only minimizes the impact of fraudulent activities but also safeguards the integrity of ad budgets. Implementing these advanced techniques in real-time threat mitigation fortifies our defenses and optimizes the overall efficacy of programmatic advertising efforts.
Frequently Asked Questions
How Do I Optimize Programmatic Ads?
To optimize programmatic ads, I focus on data segmentation, audience insights, and budget allocation. I enhance creative optimization through A/B testing, track conversions, refine ad placement, leverage real-time bidding, analyze user behavior, and monitor performance metrics.
Is Programmatic Advertising Machine Learning?
You reap what you sow. Programmatic advertising isn't just machine learning, but it's a critical component. It involves audience segmentation, campaign optimization, data integration, click prediction, bidding strategies, real-time analysis, user profiling, fraud detection, ad creatives, conversion tracking.
Can You Target Programmatic Advertising?
Yes, I can target programmatic advertising through audience segmentation, behavioral targeting, contextual advertising, geo targeting strategies, ad personalization, retargeting campaigns, demographic analysis, psychographic profiling, interest-based targeting, and cross-device tracking for precise, innovative ad delivery.
Do Ads Use Machine Learning?
Yes, ads use machine learning. By analyzing user behavior and employing click prediction, they enhance ad performance through data segmentation, contextual targeting, and audience analysis. Real-time bidding, predictive analytics, conversion tracking, and A/B testing boost effectiveness.
Conclusion
In sum, optimizing programmatic ad targeting with machine learning isn't just possible; it's revolutionary. By leveraging real-time data, refining audience segmentation, and employing predictive modeling, we can reach the right users at the right time.
Additionally, robust fraud detection mechanisms guarantee ad spend efficiency. This synthesis of machine learning and programmatic advertising transforms potential into performance, enabling smarter, more precise ad delivery.
The future of advertising isn't just near—it's now.