I've identified six top-tier predictive analytics tools that excel in various features and capabilities. Altair AI Studio stands out with extensive machine learning capabilities and automation. H2O Driverless AI offers robust model interpretability and scalability. IBM Watson Studio integrates well with Python and R and is ideal for collaborative projects. Microsoft Azure Machine Learning is lauded for its advanced forecasting and scalability. SAP Predictive Analytics leverages generative AI for enhanced forecasting. DataRobot AI Platform is recognized for its automated model-building and cloud-based flexibility. Each tool has unique strengths that can drive insightful, data-centric decisions. Discover how these tools can elevate your analytics game.
Key Takeaways
- Altair AI Studio offers extensive data science and machine learning capabilities with a user-friendly interface.
- H2O Driverless AI excels in automated machine learning and model interpretability for large datasets.
- IBM Watson Studio provides robust model development and deployment with integration support for Python and R.
- Azure Machine Learning features advanced forecasting, data visualization tools, and a scalable infrastructure.
- DataRobot AI Platform is cloud-based, offering automated model building and high accuracy in predictive models.
Altair AI Studio
Altair AI Studio stands out as a premier predictive analytics tool due to its extensive data science and machine learning capabilities. I appreciate its advanced features, which allow me to develop predictive models and conduct thorough data analysis seamlessly.
With a user-friendly interface, Altair AI Studio caters to both experts and business users, ensuring accessibility. Its automation features streamline the modeling process, while intelligent algorithm selection enhances accuracy. Leveraging these tools, I can make data-driven decisions that lead to impactful results.
The wide-ranging support for various predictive analytics tasks makes Altair AI Studio an indispensable asset in my toolkit, driving innovation and efficiency in every project I undertake.
H2O Driverless AI
When I think about H2O Driverless AI, its automated machine learning capabilities stand out, providing seamless feature engineering, model validation, and hyperparameter tuning.
The platform's model interpretability features guarantee that even complex predictive models remain transparent and understandable.
Additionally, its scalability and performance make it well-suited for handling large datasets and intricate predictive analytics tasks efficiently.
Automated Machine Learning
H2O Driverless AI revolutionizes the data science workflow by automating critical tasks such as feature engineering, model selection, hyperparameter tuning, and deployment. With automated ML, I can streamline the entire data science process, transforming complex machine learning tasks into efficient, scalable solutions.
The platform excels in predictive analytics features, ensuring high predictive model accuracy through sophisticated hyperparameter optimization techniques. Its feature engineering capabilities automatically extract the most relevant data properties, enhancing model performance.
Scalability is another strong suit, enabling me to handle diverse data sets effortlessly. Moreover, H2O Driverless AI simplifies model deployment, making the shift from development to production seamless.
This automation not only boosts productivity but also allows for more focus on innovative problem-solving.
Model Interpretability Features
Understanding the intricacies of model interpretability in Driverless AI empowers me to trust and validate the predictions made by complex machine learning models. The model interpretability features in H2O Driverless AI enhance transparency and explainability, allowing me to dissect the factors influencing predictions. By visualizing the model's decision-making process, I can identify potential biases and errors. This insight is essential for validating model performance and guaranteeing reliable AI-driven decisions.
Here's a breakdown of key interpretability aspects:
Feature | Benefit | Impact on Decision-Making |
---|---|---|
Transparency | Builds trust | Enhances confidence in outputs |
Explainability | Clarifies model workings | Simplifies complex results |
Bias Detection | Identifies potential biases | Ensures fairness |
Error Analysis | Highlights errors | Improves model accuracy |
These features collectively inform and optimize my decision-making process.
Scalability and Performance
Leveraging its robust architecture, Driverless AI seamlessly scales to handle large datasets, guaranteeing high performance and efficiency in machine learning workflows.
Its scalable performance is vital for organizations with significant data volumes.
By automating feature engineering and model selection, it accelerates machine learning processes while enhancing predictive accuracy.
The tool's automated bias detection ensures fairer and more reliable predictions, making it essential for high-performing predictive analytics.
Driverless AI's ability to manage and process extensive datasets without compromising speed or accuracy positions it as a top choice for those automating machine learning workflows.
Ultimately, its performance capabilities empower organizations to derive meaningful insights from vast amounts of data, driving innovation and competitive advantage.
IBM Watson Studio
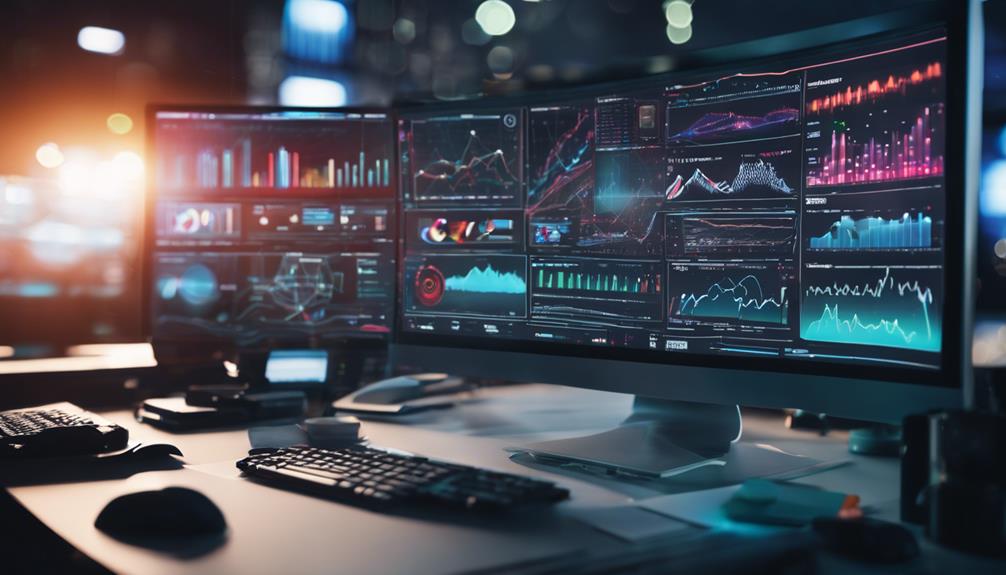
I find IBM Watson Studio to be a robust platform, offering essential tools for data preparation, model development, and deployment.
Its integration capabilities with various programming languages like Python and R make it highly versatile.
Additionally, the platform's collaboration features and wide range of machine learning algorithms add significant value for team-based projects and advanced analytics.
Key Features Overview
IBM Watson Studio excels in its wide range of features that support seamless collaboration, robust data preparation, and advanced model deployment. As a top predictive analytics tool, it harnesses AI and machine learning to improve predictive modeling. It accommodates languages like Python and R, offering flexibility in data analysis. Users benefit from pre-built models and industry-specific templates, accelerating the process of gaining insights and making predictions.
Here's a quick overview of its key features:
Feature | Description |
---|---|
Collaboration | Platform for data scientists and analysts |
Data Preparation | Tools for strong data management |
Model Building | Supports Python, R, and machine learning |
Deployment | Advanced model deployment capabilities |
Pre-built Models/Templates | Ready-to-use models for various industries |
IBM Watson Studio's extensive ecosystem ensures efficient workflows and innovative solutions.
Integration Capabilities
Building on the robust features of IBM Watson Studio, its integration capabilities stand out by enabling seamless connectivity with diverse data sources and cloud services, facilitating thorough data analysis and predictive modeling.
I find the integration capabilities particularly impressive, guaranteeing that data scientists and developers can:
- Effortlessly connect to diverse datasets for in-depth analysis.
- Utilize Jupyter notebooks and RStudio for advanced analytics and model development.
- Integrate seamlessly with IBM Cloud Pak for Data, providing a holistic data and AI platform.
- Deploy and manage machine learning models in production environments with ease.
- Foster collaboration among team members, streamlining workflows and enhancing productivity.
IBM Watson Studio's seamless integration creates an innovative environment where predictive analytics can thrive, driving impactful business insights and decisions.
Pricing and Plans
Securing the pricing and plans for Watson Studio is crucial for optimizing costs and maximizing the value of its advanced analytics features. IBM Watson Studio offers a tiered pricing structure to accommodate varying needs.
The Lite plan is free, perfect for small-scale projects or learning, providing limited features.
The Standard plan, starting at $99 per month, adds advanced collaboration tools and extra storage.
For enterprise-level requirements, the Professional plan starts at $2,000 per month, offering enhanced security, scalability, and customization options.
Pricing is usage-based, with additional charges for storage, compute resources, and add-on services, allowing users to scale according to project demands and budget constraints. This flexible approach ensures tailored solutions for innovative analytics endeavors.
Microsoft Azure Machine Learning
Microsoft Azure Machine Learning excels in delivering robust and scalable tools essential for efficient predictive analytics. This platform offers advanced AI capabilities and data analytics features, enabling users to build predictive models with remarkable accuracy. By leveraging Azure's infrastructure, users can handle large datasets and complex modeling tasks seamlessly.
- Advanced forecasting capabilities: Predict trends and outcomes with precision.
- Integrated ML and AI features: Enhance model performance and accuracy.
- Data visualization tools: Transform raw data into actionable insights.
- Scalable infrastructure: Efficiently manage extensive and complex data.
- User satisfaction: Rated 4.3 with 52 reviews, indicating reliability.
Users can build sophisticated predictive models, fostering data-driven decision-making with ease. Azure Machine Learning's capabilities in predictive analytics make it a top choice for innovators seeking powerful data solutions.
SAP Predictive Analytics
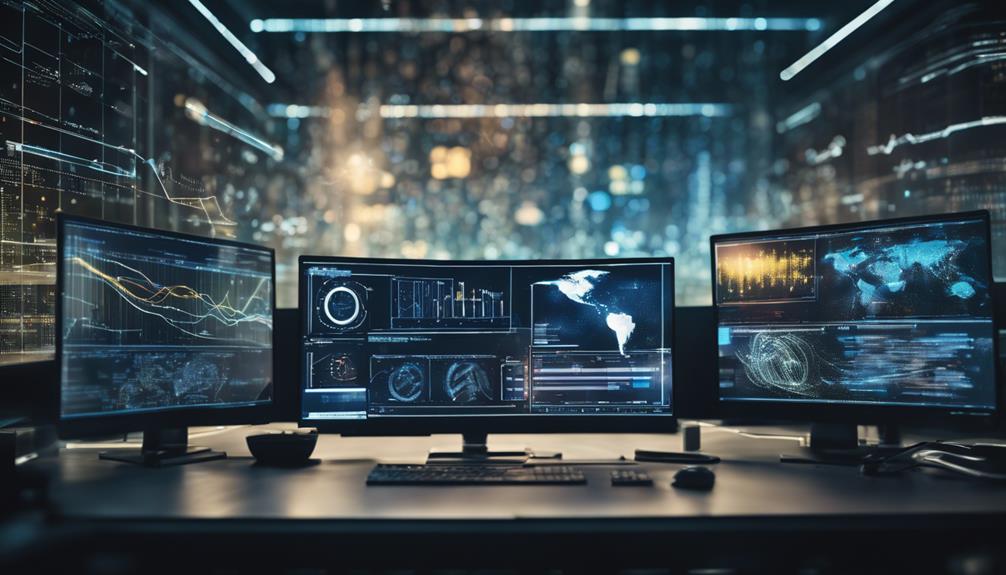
SAP Predictive Analytics stands out with its generative AI capabilities, offering a dynamic environment for interactive forecasting and diverse data sourcing. Its user-friendly platform excels at designing and deploying machine learning models, making it accessible yet powerful for users seeking data-driven insights.
Here's a glimpse of its core features:
Feature | Description | Benefit |
---|---|---|
Generative AI | Enhances forecasting with advanced AI techniques | Accurate, interactive forecasting |
Varied Data Sourcing | Supports multiple data sources for extensive analysis | Holistic data integration |
Data Visualization | Provides robust tools for visualizing intricate data | Clear, actionable insights |
Machine Learning Deployment | Facilitates easy deployment of machine learning models | Streamlined model integration |
SAP Predictive Analytics is perfect for those who need an innovative, data-centric approach to analytics.
DataRobot AI Platform
The DataRobot AI Platform revolutionizes predictive analytics with its automated machine learning capabilities, offering a streamlined, user-friendly interface that caters to both experts and non-experts. This platform accelerates the model-building process, guaranteeing high accuracy and efficiency.
As someone who values innovation, I appreciate how DataRobot supports a wide array of business uses and industries.
- Automated model-building: Saves time and improves productivity.
- User-friendly interface: Makes it accessible for all users.
- Cloud-based: Enhances scalability and flexibility.
- High accuracy: Guarantees reliable predictive models.
- Industry recognition: Validates its leadership in AI and machine learning.
Frequently Asked Questions
Which Is the Best Tool for Predictive Analysis?
I believe the best tool for predictive analysis is H2O.ai. Its cloud integration, intuitive user interface, robust data visualization, and efficient machine learning algorithms guarantee high model accuracy, seamless data preprocessing, real-time analysis, and flexible deployment strategies.
Which Statistical Tool Is Most Useful in Predictive Analytics?
When considering the most useful statistical tool in predictive analytics, I'd say regression analysis excels due to its versatility. However, decision trees, neural networks, time series forecasting, clustering methods, survival analysis, and ensemble methods also offer innovative, robust solutions.
What Are the 4 Predictive Analytics?
"Knowledge is power." In predictive analytics, machine learning, regression analysis, and time series are pivotal. Techniques like neural networks, decision trees, clustering algorithms, anomaly detection, and support vectors foster advanced predictive modeling and data mining innovations.
What Is the Most Used Technique in Predictive Analytics?
The most used technique in predictive analytics is machine learning. It encompasses data mining, regression analysis, decision trees, neural networks, ensemble methods, feature selection, time series, and clustering techniques, excelling in pattern recognition and innovative solutions.
Conclusion
After diving into the world of predictive analytics tools, it's clear that each platform offers its own unique blend of power and finesse. Altair AI Studio, H2O Driverless AI, IBM Watson Studio, Microsoft Azure Machine Learning, SAP Predictive Analytics, and DataRobot AI Platform can turn the murky waters of raw data into crystal-clear insights.
Choosing the right one depends on your specific needs, but rest assured, with any of these, you're in capable hands.