I recommend starting by automating repetitive tasks with clear rules and significant data input. Prioritize thorough data cleansing and robust preparation for better results. Selecting the right AI tools that align with organizational needs and feature easy integration is essential. Pilot projects help validate AI effectiveness on a smaller scale. Real-time data analysis aids in optimizing and refining processes continuously. Transparency through clear data logging guarantees accountability. Lastly, active stakeholder collaboration streamlines decision-making and aligns AI initiatives with business goals. To uncover all the strategic details and refined approaches, keep exploring further.
Key Takeaways
- Focus on automating repetitive tasks with clear rules to maximize efficiency.
- Prioritize data preparation and cleansing to ensure robust and reliable AI models.
- Choose AI tools with scalability, budget-friendliness, and advanced capabilities like OCR and workflow automation.
- Start with pilot projects on data-intensive tasks to validate effectiveness and feasibility.
- Continuously monitor performance metrics and real-time data to refine and optimize processes.
Identifying Tasks for Automation
When identifying tasks for automation, we should zero in on repetitive tasks with clear rules and minimal human judgment.
Implementing AI in our workflow can transform how we manage business processes. To effectively use AI workflow automation, we need to target tasks that involve high data input/output and significant time consumption.
These tasks are prime candidates for process automation due to their repetitive nature and low complexity. By automating these areas, we can streamline operations and reduce costs.
Focusing on departments with low productivity or areas with high data volume guarantees we're maximizing the impact of AI.
Ultimately, selecting the right tasks to automate lays a strong foundation for efficient and innovative business processes.
Preparing and Training Data
After pinpointing the tasks suited for automation, the next step is ensuring our data is meticulously prepared and trained to maximize AI effectiveness.
First, we need to prioritize data cleansing and data preparation to guarantee high-quality inputs for our algorithms. Techniques like feature engineering and normalization are vital for enhancing model performance. We should leverage data visualization platforms and data preprocessing libraries for efficiency.
Continuous monitoring of our data training processes is essential to maintain the effectiveness of our AI models. Here's a strategic approach:
- Data Cleansing: Remove inaccuracies and inconsistencies.
- Feature Engineering: Create relevant features to improve model accuracy.
- Monitoring Data Training: Regular updates ensure reduced bias and enhanced predictive capabilities.
This ensures our data sets remain robust and reliable.
Selecting Suitable AI Tools
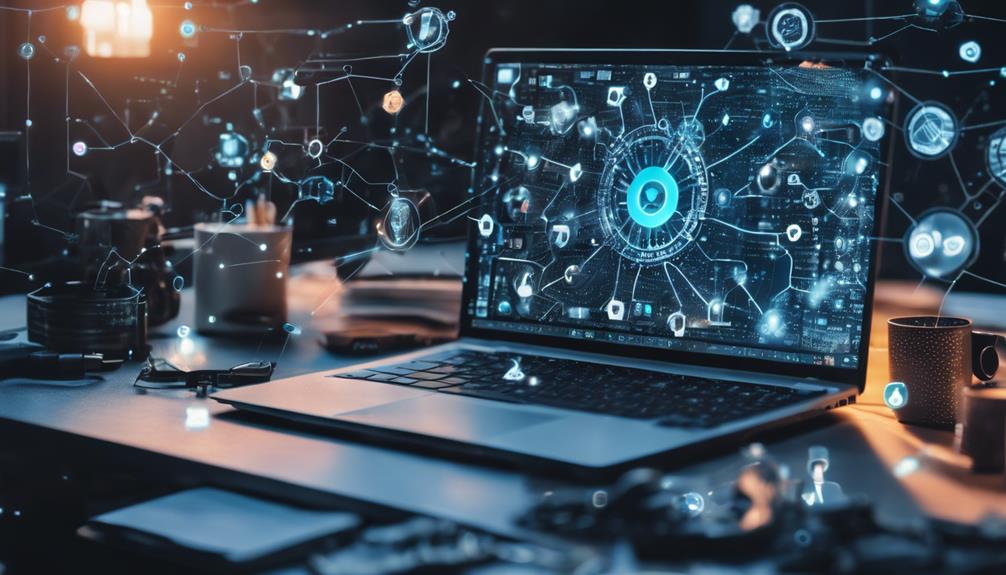
Selecting suitable AI tools starts with evaluating their scalability, budget-friendliness, and advanced capabilities like machine learning and natural language processing.
Tools such as Zendesk, Atlassian, and HubSpot should be on your radar for their chatbots and workflow automation features.
Look for AI tools that excel in OCR, generative AI, and ease of integration with existing systems. These capabilities are crucial for enhancing operational efficiency, intelligent document processing, and overall workflow automation.
Ensuring a smooth user experience is essential for maximum adoption. By aligning AI tools with your organization's specific needs, you can eliminate busy work and greatly improve operational efficiency.
Choose wisely and strategically to maximize the benefits of AI-enhanced workflow automation.
Starting With Pilot Projects
Once you've identified the right AI tools, it's prudent to start with pilot projects to validate their effectiveness and feasibility in your workflows. Starting small by testing AI-driven workflow automation on a limited scale minimizes risks and costs.
Pilot projects enable you to identify suitable tasks for automation, providing valuable insights into the scalability and adaptability of AI solutions.
Here's a strategic approach:
- Choose Suitable Tasks: Focus on tasks that are data-intensive and repetitive.
- Set Clear Objectives: Define what success looks like regarding feasibility and effectiveness.
- Analyze Pilot Projects Insights: Gather data to understand user acceptance and potential scalability.
Monitoring and Optimizing Processes
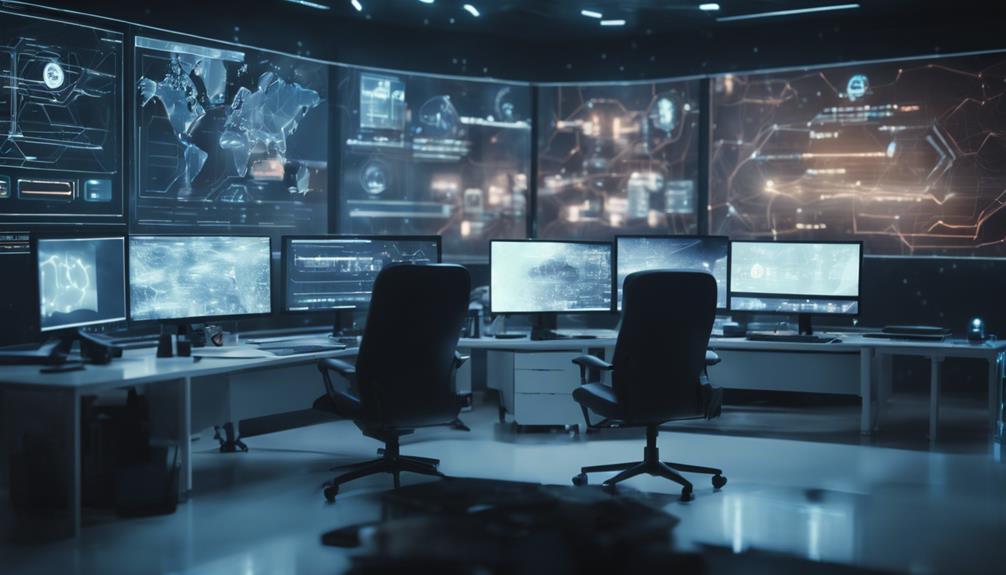
When I monitor AI-enhanced workflows, real-time data analysis is essential for tracking performance and identifying inefficiencies.
By evaluating performance metrics, I can pinpoint areas needing optimization and adjust algorithms accordingly.
This strategic approach guarantees that our workflows remain efficient and continuously improve.
Real-time Data Analysis
Leveraging real-time data analysis in AI-enhanced workflow automation empowers organizations to continuously monitor and optimize their processes for immediate insights and adjustments. By incorporating AI-driven monitoring, we can identify bottlenecks, detect anomalies, and recognize trends as they happen. This facilitates proactive responses, ensuring enhanced operational efficiency.
Here's how real-time data analysis can transform your workflows:
- Agile decision-making: Quickly adapt to changes, making informed decisions based on current data.
- Resource allocation: Optimize the use of resources by identifying where they're most needed and reallocating them efficiently.
- Enhanced performance: Continuously refine processes to reduce errors and improve overall performance.
Embracing real-time data analysis in workflow automation is key to staying competitive and innovative.
Performance Metrics Evaluation
By systematically evaluating performance metrics, we can guarantee our AI-enhanced workflow automation not only adapts in real-time but also continuously improves.
Monitoring key metrics like process completion time, error rates, and resource utilization allows us to conduct thorough efficiency evaluations. Analyzing task completion rates and response times with advanced analytics tools aids in identifying bottlenecks and making data-driven decisions for optimization.
By comparing pre-automation and post-automation metrics, we measure AI's impact on productivity gains and cost savings. Utilizing real-time data insights ensures our workflows align with business goals, enhancing overall performance.
Continuous optimization of AI-enhanced workflows is essential for maintaining peak efficiency and responsiveness in dynamic environments.
Ensuring Transparency and Accountability
To guarantee transparency and accountability in AI-enhanced workflow automation, I focus on clear data logging and robust audit trail management.
By implementing Explainable AI techniques, I provide clear reasons behind AI decisions.
Additionally, regular audits and stakeholder feedback are essential for maintaining trust and reliability in the system.
Clear Data Logging
In AI workflow automation, clear data logging plays an essential role in ensuring transparency and accountability by meticulously recording every action the system takes. This thorough data logging captures input data, processing steps, and output results, establishing a robust audit trail.
Here's why it's vital:
- Error Identification: Pinpointing errors within automated processes becomes straightforward, enabling swift rectifications and minimal disruptions.
- Compliance: Maintaining a detailed log aids in meeting regulatory requirements and adhering to internal policies, showcasing responsible use.
- Traceability: Clear data logging ensures every action is traceable, fostering transparency and boosting trust in AI systems.
Audit Trail Management
Effective audit trail management captures every user activity, system event, and data change, guaranteeing transparency and accountability in AI-enhanced workflows. By meticulously tracking these elements, I can maintain compliance and traceability, meeting stringent regulatory requirements.
Thorough audit trails enable me to identify bottlenecks and analyze performance metrics, thereby enhancing process efficiency. Clear policies and procedures ensure data integrity and protect against fraud. Audit trail reports offer vital insights into the workflow, facilitating strategic decisions and continuous improvement.
This robust management approach doesn't just meet legal obligations; it also builds trust and reliability in automated systems. Embracing audit trail management is essential for sustaining innovation while ensuring transparency and accountability in every automated process.
Collaborating With Stakeholders
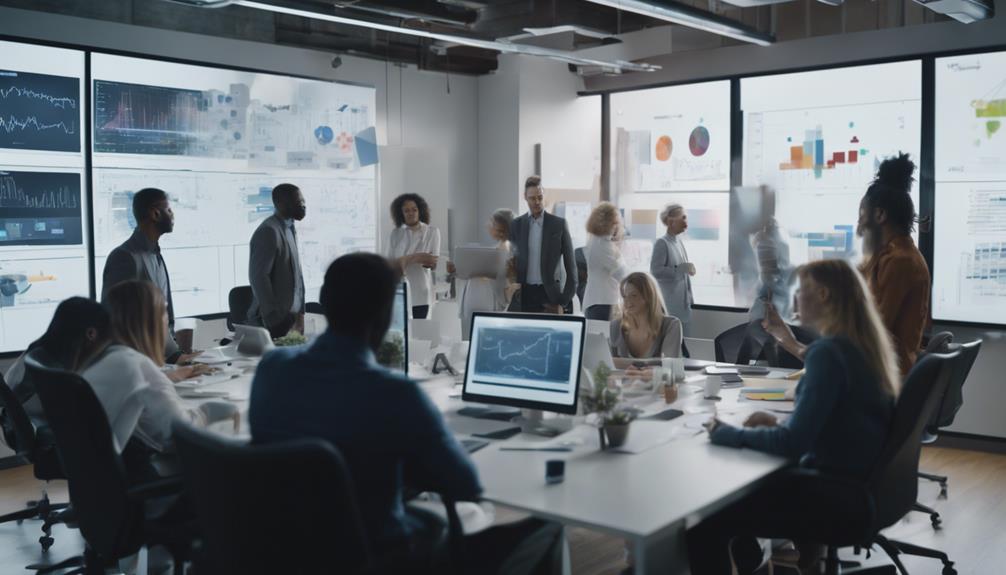
Engaging stakeholders early allows us to gather critical input on workflow automation needs and challenges, ensuring our AI objectives align with business goals. By collaborating closely with stakeholders, we foster alignment and streamline the decision-making process for our automation initiatives.
It's important to seek continuous feedback throughout the implementation process to address concerns effectively and make necessary adjustments.
Here's how we can enhance stakeholder collaboration:
- Active Participation: Encourage stakeholders' active participation in discussions to refine automation strategies.
- Open Communication: Maintain transparent communication channels to keep all parties informed and engaged.
- Feedback Integration: Regularly incorporate stakeholder feedback to fine-tune the implementation process.
Effective collaboration drives successful AI-enhanced workflow automation, ensuring our strategies are robust and well-supported.
Frequently Asked Questions
How to Use AI to Improve Workflow?
I use AI to improve workflow by leveraging predictive analytics for task prioritization, data integration for process optimization, employee training, workflow monitoring, resource allocation, compliance management, customer support, and enhancing decision-making for strategic, efficient operations.
What Are the Four Stages of an AI Workflow?
The four stages of an AI workflow are monumental! First, Data Collection, then Data Preprocessing, followed by Model Training with Algorithm Selection and Feature Engineering. Finally, Model Deployment involves Performance Monitoring, Error Analysis, Result Interpretation, and tackling Scalability Challenges.
What Are the 5 Steps of Workflow?
I define the process with process mapping, assign tasks, allocate resources, and set deadlines. I track progress using performance metrics, conduct bottleneck analysis, prioritize tasks, and standardize workflows. Continuous feedback and team coordination drive efficiency improvements.
What Is AI Workflow Automation?
Imagine using a crystal ball: AI workflow automation leverages machine learning, predictive analytics, and smart algorithms. It utilizes robotic automation, intelligent agents, cognitive computing, and workflow intelligence for task automation and process optimization, driving efficiency improvement and innovation.
Conclusion
So, there you have it—automate your way to utopia by following these 'simple' steps. Identify tasks? No problem! Train data? Piece of cake! Select AI tools like a tech wizard, kick off pilot projects, and voila, you're practically a magician.
Monitor, optimize, and guarantee transparency? Easy, right? Just remember to keep everyone in the loop, because who doesn't love a perfectly orchestrated symphony of AI and human collaboration?
Good luck, future automation overlord!